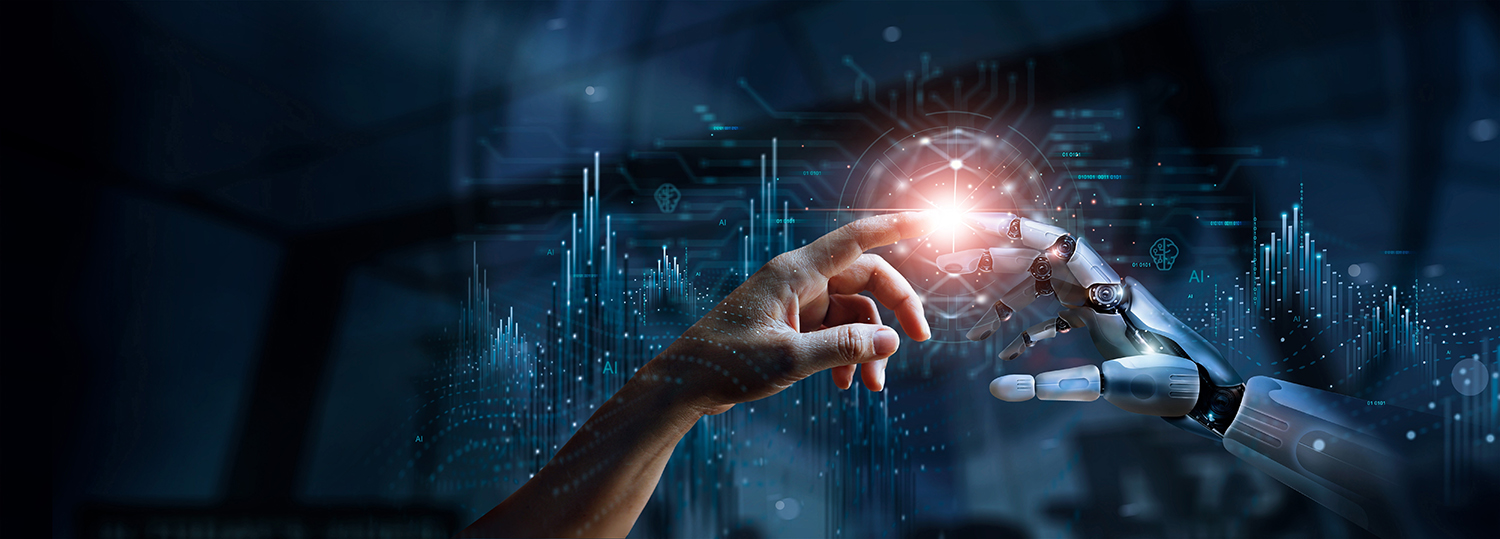
Applied AI in Engineering & Computer Science Symposium
2024 Symposium Recap
On Wednesday, Sept. 18, the Applied AI in Engineering & Computer Science Symposium brought together industry leaders and NC State College of Engineering faculty, staff and students for discussion on our world’s AI-driven future and what the college can do now to ensure our students are prepared.
Videos of the keynote speakers and industry roundtable are available below, as are event photos and the 90 posters that were presented by the college’s talented graduate students.
This is an exciting time for the college. Faculty members and graduate students are already using AI in their research, but there is more the college can do to integrate AI into curricula across engineering and computer science disciplines and to prepare students for an ever-shifting technology landscape.
Dean Pfaendtner’s Takeaways
There is no better time for our college to become a preeminent center for applied AI in engineering and computer science.
With our expansion through Engineering North Carolina’s Future, now is the time to hire faculty members with expertise in applying AI to their research and teaching. Our department heads are on board with this strategy. Beyond hiring, we are thinking critically about what the classrooms and labs of the future will look like in our new engineering building.
We can’t yet see the full view of where applied AI will take us. But we do know data-driven engineering is going to be a critical part of the future.
Even the leading experts don’t know exactly how AI will change our industries. What we need to focus on in our College of Engineering is ensuring our students are skilled in data-driven engineering and that they are comfortable navigating an ever-changing technology landscape.
Collaboration and communication are two important skills our students need to develop.Almost every speaker emphasized collaboration and communication as critical skills needed to build and use AI interfaces effectively. Our students need to know how to clearly share ideas, details and context not just while developing AI technologies, but also when talking and listening to end users.
Our faculty, staff and graduate students are already working on the cutting-edge in applying AI to their research.
We have a strong base to build off of, and the breadth and depth of research on display was inspiring. Our faculty members and students are creatively applying AI to so many disciplines. Posters mentioned just about anything you could think of: guide dogs, DNA storage, robotic limbs, historical Arabic documents, yams, autonomous vehicles, materials discovery and so much more.
AI is a key tool in making learning more effective and engaging.
One-on-one tutoring and narrative-centered learning are two of the most effective learning experiences. AI is a powerful tool that can scale up the use of both in classrooms to help students remain engaged and motivated, and I am excited to see our continued developments in this space.
Investors are interested in AI, but a lot of work needs to be done to ensure infrastructure is ready.
There needs to be a massive shift to increase our global data storage capacity so that all industries and academic settings can embrace and integrate AI into their work. As LLMs took off, it put more and more pressure on our power grid and resources. Part of moving toward an AI-driven future is ensuring we have the infrastructure to support it. Our college has been working for more than a decade to develop the power grid of the future through the FREEDM Systems Center.
Videos
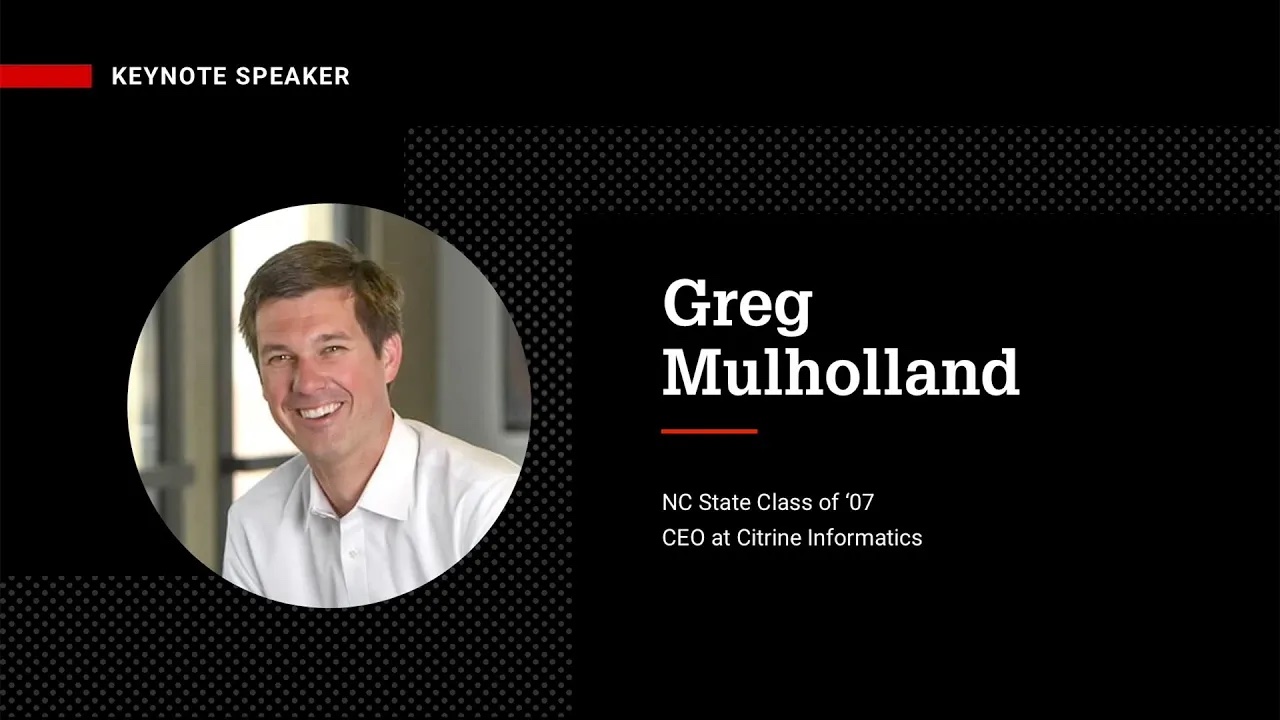
Applied AI in Engineering and Computer Science Symposium: Greg Mulholland
Watch now
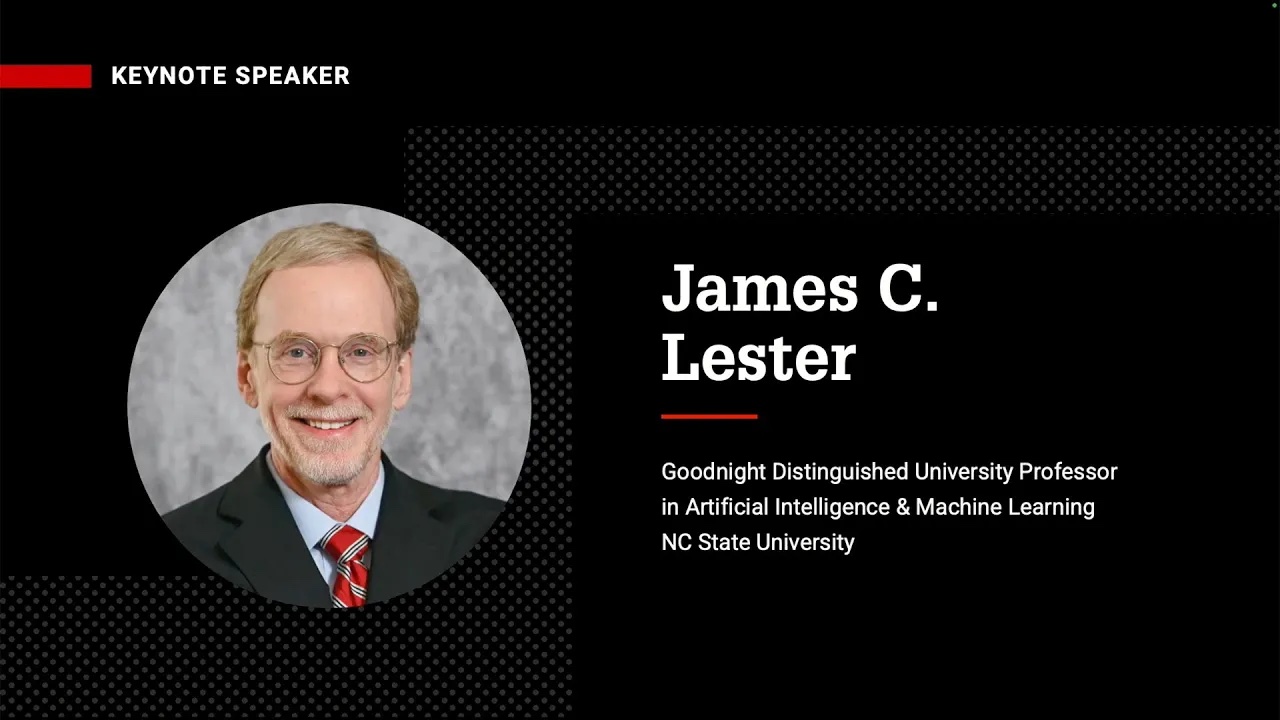
Applied AI in Engineering and Computer Science Symposium: James Lester
Watch now

Applied AI in Engineering and Computer Science Symposium: Radhika Venkatraman
Watch now
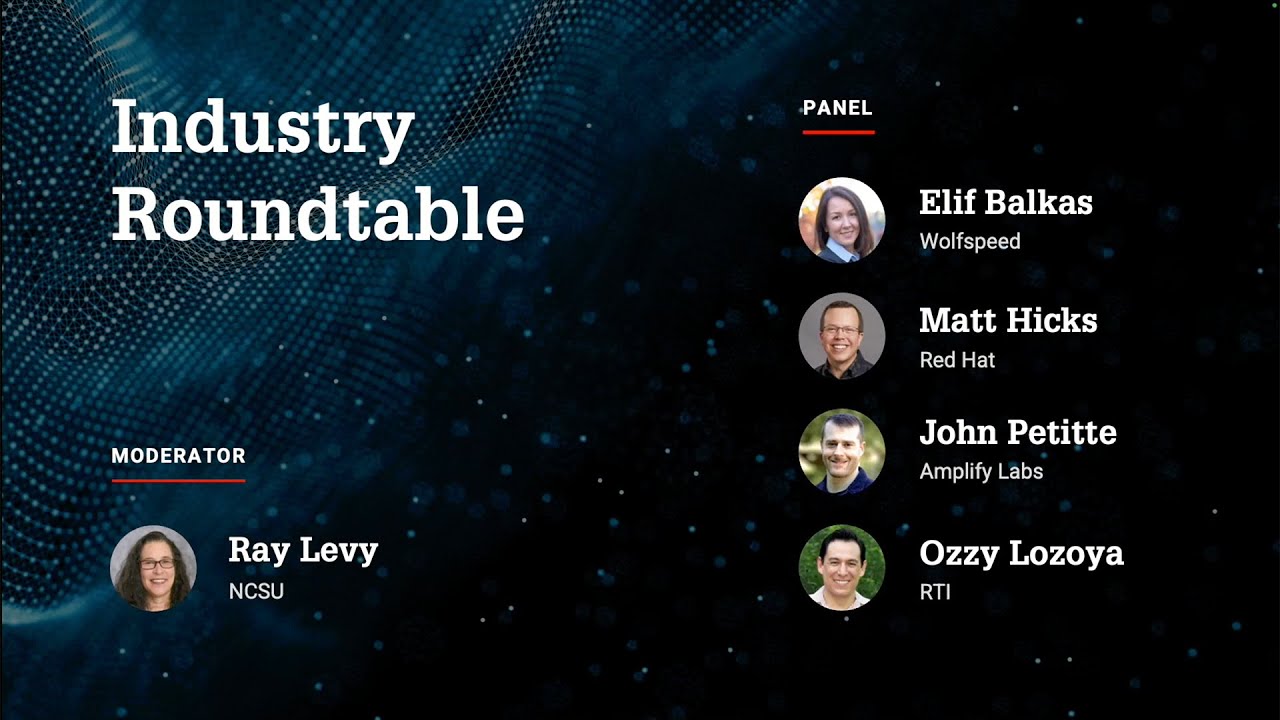
Applied AI in Engineering and Computer Science Symposium: Industry Roundtable
Watch now
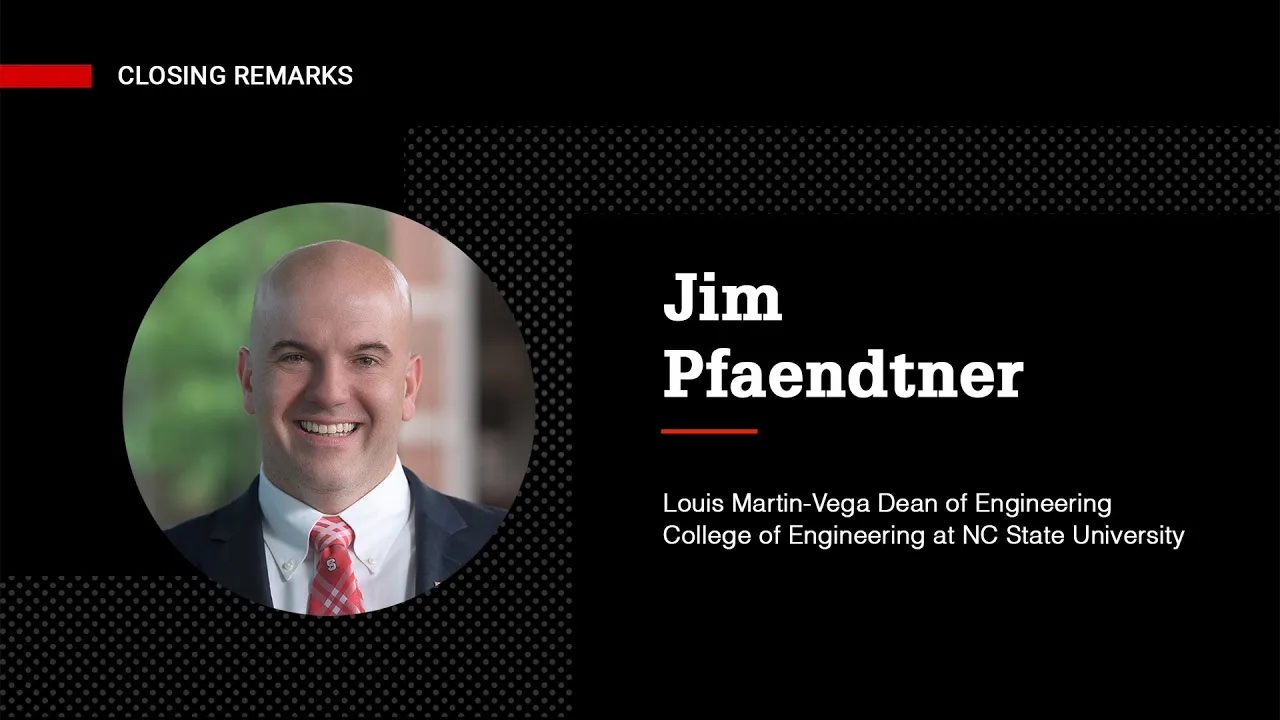
Applied AI in Engineering and Computer Science Symposium: Jim Pfaendtner
Watch now
Photo Gallery
Advisory Committee
Poster Session
The 2024 Applied AI in Engineering & Computer Science Symposium poster session provided College of Engineering faculty, postdoctoral researchers, and graduate students an opportunity to learn more about the breadth of AI-related research currently underway in the college.
We encouraged posters that demonstrate the applications of AI in interdisciplinary areas and cover a broad spectrum of applications and AI tools utilized.
The Graduate Student Poster Session featured College of Engineering graduate students currently enrolled in an engineering program or postdoctoral researcher conducting research in an AI-related field or using AI to advance research.
Click on the following buttons to jump to the academic department of your choice.
Biological and Agricultural Engineering
Shana McDowell
smmcdow2@ncsu.edu
Faculty Sponsor: Daniela Jones
Project Title: The Development of Machine Learning Models for the Assessment of In-season Sweetpotato Root Growth and Crop Yield Estimates
Project Abstract
The Development of Machine Learning Models for the Assessment of In-season Sweetpotato Root Growth and Crop Yield Estimates
Sweetpotatoes vary widely in shape and size, and consumers prefer particular characteristics over others. Optimizing the yield to match consumer preferences would increase grower’s profits and reduce waste. We used machine learning algorithms to highlight the environmental factors, harvesting, planting, and application decisions that contribute to sweetpotato shape and size throughout the growing season. In this process, we also gained a better understanding of the sweetpotato developmental stages through root images of sweetpotatoes as input into computer vision algorithms. We worked with a large sweetpotato grower, packer, and distributor in North Carolina that manages nearly fifteen thousand acres. The provenance of data collected for this study is from several on-farm locations scattered over a geographical area of about 2,500 square miles and from two growing seasons. Sweetpotato weight and length-to-width ratio were predicted using Linear Regression, Support Vector Machine, Random Forest, and XG Boost models. Results show that important predictors of sweetpotato weight and length-to-width ratio are: minimum temperature, soil bulk density, and cumulative precipitation. Elevation, fertilizer application also came up as an important variable for sweetpotato length-to-width ratio. When predicting average weight, the Linear Regression model performed the best (RMSE =4.44, R2 of 0.49) and the Random Forest performed best when predicting Length-to-width ratio (RMSE= 0.44, R2 of 0.74). As part of our translational research efforts, we have also created a dashboard that displays in-field data and supports stakeholder decision-making throughout the growing season to determine an optimal harvest window. Growth estimates obtained from this analysis will be compared to growth measurements of previous growing seasons. This analysis will aid in the development of farm-to-market decision models resulting in a data-driven agri-food supply chain, an optimized crop yield, and a better use of grower’s resources.
Leticia Santos
lsantos2@ncsu.edu
Faculty Sponsor: Daniela Jones
Project Title: Crop residue cover mapping by integrating multi-year satellite imagery and machine learning
Project Abstract
Crop residue cover mapping by integrating multi-year satellite imagery and machine learning
Climate smart agricultural practices focus on farm management techniques that can improve soil health, such as conservation tillage. Conservation tillage leaves crop residue on the soil surface after harvest, which acts as a protective layer against erosion, conserving soil moisture by shielding against extreme heat, and suppressing weeds. Currently, the most accurate method used to assess conservation tillage adoption is through ground-level surveying, which, although effective, is time-consuming, labor-intensive, and not easily scalable. Remote sensing allows ground surveys to be scaled up to estimate crop residue cover over larger extents. Crop residues, characterized by high lignocellulose content, are best differentiated using narrow-band shortwave infrared (SWIR) bands that are available in Worldview 3 (WV3) imagery. While WV3 imagery has high spatial resolution (~ 3.5 m in the SWIR), imagery is not publicly available, is only collected when tasked, and the resultant images only cover a small spatial footprint. In contrast, publicly accessible Landsat and Sentinel imagery covers larger spatial extents, but only have two broad SWIR bands , which cannot measure lignocellulose absorption directly due to their placement. In this study, we used high-accuracy crop residue cover maps derived from WV3 imagery and ground survey data to train machine learning techniques including random forest, gradient boosting, and neural networks to estimate crop residue cover with moderate-resolution Harmonized Landsat Sentinel-2 (HLS) imagery. We calculated various spectral indices derived from HLS bands to estimate fractional crop residue cover on the Delmarva Peninsula, USA for 2017, 2019, 2021 and 2022. All of the machine learning methods tested were effective for predicting crop residue cover, with RMSE lower than 0.1, for all years. The lowest RMSE was obtained with a 3-layer (16 neurons per layer) neural network, using the 2022 year, which is likely related to the close temporal pairing of the HLS and WV3 image acquisitions. This modeling approach will enable region-scalable estimation of crop residue cover that could be used to more accurately monitor conservation tillage adoption on agricultural lands.
Biomedical Engineering
Matt Dausch
medausch@ncsu.edu
Faculty Sponsor: David Lalush
Project Title: Anatomical Standardization of Magnetic Resonance Images of the Knee Using AI-Based Segmentation and Two-Channel Deformable Registration
Project Abstract
Anatomical Standardization of Magnetic Resonance Images of the Knee Using AI-Based Segmentation and Two-Channel Deformable Registration
Introduction
Magnetic resonance (MR) imaging is a common tool used to study Osteoarthritis (OA) due to its high soft tissue contrast and its ability to effectively visualize affected cartilage. Traditionally, MRI-based studies have segmented images into regions of interest (ROIs) or utilized anatomical standardization of a population of images to identify statistically significant ROIs. Anatomical standardization, such as the use of brain templates for functional MRI experiments, is generally preferred if the ROI is not necessarily confined to investigator predefined ROIs. The knee has proved to be slightly difficult to standardize due to greater variations in anatomy between individuals. In this study, we propose a two-channel method of constructing an anatomical knee template utilizing both an anatomical MR knee image and a label image segmenting the tibia and femur via a U-net convolutional neural network to facilitate the goal of a finer grained analysis of the cartilage surface.
Image Acquisition
Anatomical and T1rho MR knee images were taken with a double echo steady state (DESS) sequence and spin-locked T1rho sequence respectively on a 3T Magnetom Prisma (Siemens) during the same scan session.
Bone Labeling
The anatomical DESS knee images were used to label the tibia and femur. This labeling was done via a 55-layer U-net image segmentation network trained on 33 investigator-labeled DESS knee images.
Knee Template
The knee template was created as an anatomical average of 21 DESS uninjured knee images using Advanced Normalization Tools (ANTs) (University of Pennsylvania). The creation of the template utilized two-channel images, the anatomical DESS image and the bone-label image, in a greedy B-spline symmetric image normalization (SyN) deformable registration through five iterations.
Registration of Individual Knee to Two-Channel Template
DESS registration of an individual subject’s knee to the template used ANTs in a three-step transformation process (rigid, affine, deformable) to determine a deformable mapping from its intrinsic coordinate system to the template coordinate system. Four T1rho relaxation time volumes were then warped with the calculated transform to fit the template then used to calculate a quantitative T1rho relaxation time map in the template coordinate system.
Results/Discussion/Conclusion
When the final quantitative T1rho map is fully warped, it mimics the anatomical structure of the template with the DESS image resolution. The most consistent and clean warping of the cartilage is at the bottom of the femoral condyle and the top of the tibial condyle while cartilage located towards the anterior/posterior sides of the femoral condyle resulted in slightly more blurring in the template. T1rho maps were able to be successfully warped in most cases to match the cartilage anatomy to that of the template despite the differences in intra-slice (0.4375mm x 0.4375mm to 0.3125mm x 0.3125mm) and inter-slice (4mm to 1mm) resolution. The method of transforming and averaging a sample set of MR knee images and bone labels created a two-channel knee template allowing for the anatomical standardization of an individual subject’s knee. This standardization ultimately allows for the future goal of creating a finer-grained analysis of the cartilage surface over a population.
Krysten Lambeth
kflambet@ncsu.edu
Faculty Sponsor: Nitin Sharma
Project Title: Dynamic Mode Decomposition with Sonomyography and Electromyography for Modeling Exoskeleton Walking
Project Abstract
Dynamic Mode Decomposition with Sonomyography and Electromyography for Modeling Exoskeleton Walking
The nonlinear dynamics required to model human-exoskeleton walking present a high computational burden that makes real-time model-based optimal control difficult. To address this problem, we use extended dynamic mode decomposition to approximate the Koopman operator. This allows us to derive a linearized model of the human-exoskeleton system. Treadmill data during exoskeleton-assisted walking are collected from participants with and without spinal cord injury and used to generate single-leg walking models. The control inputs to the model include EMG and ultrasound-derived muscle activation metrics in addition to normalized motor currents. Ground reaction force is included during the stance phase as a disturbance. We compare models which use different combinations of EMG and ultrasound-derived metrics and assess the impact of including ground reaction force on model accuracy. Accuracy was quantified by calculating the NRMSE of each state (thigh and shank angles and angular velocities) across the entire data collection period and taking the mean across states.
I-Chieh Lee
ilee5@ncsu.edu
Faculty Sponsor: Helen Huang
Project Title: Effective and Efficient Personalization of Musculoskeletal Models Using Reinforcement Learning to Inform Physical Rehabilitation and Assistive Device Control
Project Abstract
Effective and Efficient Personalization of Musculoskeletal Models Using Reinforcement Learning to Inform Physical Rehabilitation and Assistive Device Control
Musculoskeletal models have been important tools in physical rehabilitation and assistive device control. These models involve many parameters, such as muscle length, maximum contraction force, etc., to operate appropriately. Researchers have built generic musculoskeletal models based on measurements from cadavers or data collected from many individuals without physical disabilities. Due to large inter-human variations, particularly in people with motor deficits, personalizing these models is necessary. Our group developed a novel framework that efficiently personalizes electromyography (EMG)-driven musculoskeletal models. The framework adopts a generic upper-limb musculoskeletal model as a baseline and uses an artificial neural network-based policy to fine-tune the model parameters for musculoskeletal model personalization. The policy was trained using reinforcement learning (RL) to heuristically adjust the musculoskeletal model parameters, maximizing the accuracy of estimated hand and wrist motions from EMG inputs. The results of human-involved validation experiments showed that, compared to the baseline generic musculoskeletal model, personalized models estimated joint motion with lower error in both offline (p < 0.05) and online tests (p = 0.014), underscoring the importance of model personalization. The RL-based framework was three orders of magnitude faster in tuning the model for optimized motion estimation compared to a traditional global search-based optimization method. This study presents a novel concept that combines human physical models and AI to transform generic models into user-specific ones effectively and efficiently. The personalized musculoskeletal models will be applied to inform stroke rehabilitation or neural control of prosthetic arms in the future.
Varun Nalam
vnalam@ncsu.edu
Faculty Sponsor: Helen Huang
Project Title: Inverse reinforcement learning based control for human-robot symbiosis in wearable robotics
Project Abstract
Inverse reinforcement learning based control for human-robot symbiosis in wearable robotics
Wearable robotics hold immense promise in restoring mobility for patients with disabilities stemming from amputations or neurological disorders. These devices offer the potential to significantly enhance the quality of life by enabling more natural and efficient movement. However, a major challenge in the field is the seamless integration of robotic systems with the human user, whose performance is often compromised. Achieving appropriate control of the robot is crucial to ensure that the device functions as an extension of the user’s body. Reinforcement learning (RL) based control has been successfully implemented and demonstrated in both prosthesis and exoskeletons, allowing for the personalization of robotic control to meet the unique needs of each individual user. Despite its successes, a critical aspect of RL in wearable robotics is the formulation of an appropriate cost function. The cost function guides the learning process by defining what constitutes “”success”” for the robotic control system, and in the context of human-robot interaction, this is particularly complex. The dynamic and intricate interactions between the human and the wearable robot make it difficult to establish a cost that is both practical and sufficiently representative of the user’s needs and goals.
To address this challenge, we proposed a bilevel optimization-based control approach that leverages inverse reinforcement learning (IRL) to determine the most appropriate cost function for each user. This inferred cost function is then used to guide the reinforcement learning process, enabling the robot to personalize its behavior according to the user’s specific requirements. We have implemented this approach on a lower limb prosthesis, demonstrating its effectiveness not only in restoring the function of missing joints but also in strengthening the weak residual joints of individuals with amputations. By accurately modeling the user’s preferences and optimizing the control strategy accordingly, our approach enhances the overall integration of the wearable robot with the user’s body. Ultimately, this method has the potential to significantly improve the autonomy and mobility of individuals with disabilities, offering them a higher degree of independence and a better quality of life.
Jimmy Tabet
jimmy_tabet@med.unc.edu
Faculty Sponsor: Shawn Gomez
Project Title: AI-Driven Innovations in Biomedical Research, Therapy Design, and Clinical Decision Support
Project Abstract
AI-Driven Innovations in Biomedical Research, Therapy Design, and Clinical Decision Support
In the Gomez Lab, our research focuses on developing artificial intelligence (AI) solutions to augment and enhance clinical decision-making across various biomedical domains, with the end-goal of enhancing patient outcomes. Our work applies AI across various areas, spanning from diagnostic tools to the rational design of novel therapies.
One example of our work is in the development of AI models to assist in breast cancer surgery. For instance, we have created deep learning models that analyze intraoperative specimen mammography images to predict whether tumor margins are clear of cancer cells. These models aim to help surgeons make more informed decisions during surgery, while still in the operating room, reducing the need for additional procedures and improving patient outcomes.
In addition to diagnostic tools, we are exploring more fundamental AI model development. As an example, we are working to improve knowledge representation approaches and have developed a Semantically-Aware Latent Space Autoencoder (SALSA) for drug discovery and development. SALSA is a novel methodology that enhances molecular representation in deep learning models by incorporating structural similarities between molecules into the model. This approach has the potential to improve the accuracy and relevance of predictions of how new drug compounds will interact at a molecular level and for rapid chemical similarity search. The underlying methodology can also be generalized to the study of other network structures such as cell signaling networks in disease for which we have developed other deep learning methodologies.
Furthermore, we are investigating the use of machine learning to predict how targeted therapies such as kinase inhibitors–a class of drugs increasingly used in cancer therapy–will affect different cancer cells and the surrounding tissue, either alone or in combination. By integrating data from large-scale kinome profiling and gene expression studies, our models can identify promising therapeutic regimes that are entirely novel or may have been overlooked by traditional empirical methods. These computational tools offer a more efficient pathway to developing effective cancer treatments while reducing toxicity and other undesired effects.
In summary, our research aims to bridge the gap between AI methodologies and practical applications in the clinic. We believe that our work has the potential to significantly impact clinical practice, from improving diagnostic accuracy to accelerating the discovery of life-saving therapies. We look forward to collaborating with others in the AI community to further these goals and to contribute to the development of integrated, AI-driven healthcare solutions.
Xiangming Xue
xxue5@ncsu.edu
Faculty Sponsor: Nitin Sharma
Project Title: Enhancing Tremor Suppression via AI-Driven Data Modeling and Afferent Stimulation Control
Project Abstract
Enhancing Tremor Suppression via AI-Driven Data Modeling and Afferent Stimulation Control
This project focuses on advancing tremor suppression techniques through the integration of artificial intelligence (AI) and ultrasound (US) technology, specifically targeting individuals suffering from Parkinson’s Disease (PD) and Essential Tremor (ET). By harnessing the power of Dynamic Mode Decomposition (DMD) within a data-driven modeling framework, we aim to refine and validate a system that not only predicts tremor dynamics with high precision but also actively controls tremor through afferent muscle stimulation (AMS).
Central to our approach is the use of a personalized AI-enhanced model that incorporates data from US sensors to continuously monitor and analyze muscle activity. This model leverages DMD to extract dynamic patterns from high-frequency US data, thus providing a novel method for understanding tremor behaviors in real-time. Coupled with this, we employ Koopman-based system identification and a model predictive control (MPC) scheme to adjust stimulation parameters dynamically, responding to the patient-specific tremor characteristics observed.
The primary hypothesis of our research is that this multi-faceted, AI-driven model significantly improves the accuracy of tremor predictions compared to conventional methods. Moreover, it optimizes the effectiveness of afferent stimulation protocols used to suppress tremors. We anticipate that our AMS control strategy, which allows for the precise tuning of stimulation based on real-time data, will provide superior tremor suppression outcomes.
In preliminary experiments, we employed this model to simulate tremor dynamics and control in a clinical setting. Using US imaging to capture muscle movements and an inertial measurement unit (IMU) to record wrist motions, we developed comprehensive profiles of tremor behaviors under various conditions. These profiles were then used to train our model, enhancing its predictive capabilities. Notably, our findings have shown a significant reduction in normalized root mean square errors (NRMSE) for both wrist angle and angular velocity predictions when using our AI-driven model, with improvements compared to traditional one-step prediction models. This research not only underscores the potential of AI in medical diagnostics and treatment but also highlights the interdisciplinary application of AI technologies in enhancing quality of life for individuals with movement disorders.
This validation study not only seeks to affirm the efficacy of our innovative model but also to demonstrate its scalability and adaptability in real-world clinical settings. By successfully marrying AI with clinical practices, we aim to set new standards in the treatment of tremors, providing a robust, reliable, and patient-centered solution that could be adapted for a range of neuromuscular conditions.
This project exemplifies the transformative potential of AI in healthcare, particularly in the development of personalized medicine and the management of chronic conditions. It is poised to offer significant contributions to health informatics, biomedical engineering, and neuroengineering, promising to revolutionize how tremor and other movement disorders are treated in clinical environments.
Chemical and Biomolecular Engineering
Jeffrey Bennett
jabenne4@ncsu.edu
Faculty Sponsor: Milad Abolhasani
Project Title: Autonomous Catalyst Optimization for In-Flow Hydroformylation
Project Abstract
Autonomous Catalyst Optimization for In-Flow Hydroformylation
Machine learning (ML) and flow chemistry are being leveraged in chemical reaction engineering to optimize complex processes as well as to reduce time-to-discovery and enhance development. Recently, there has been a sizable increase in the use of ML algorithms to both optimize and model complex reaction systems. ML algorithms can help to rapidly identify patterns in high-dimensional reaction spaces, predict reaction outcomes, and optimize operating conditions. These data-driven approaches help to effectively leverage the high-throughput data generated by self-driving lab approaches.
One of the primary applications of ML in reaction engineering is in the development of predictive response models. These models can be used to form a digital twin of the chemical reaction and predict process outcomes, reducing the number of experiments needed to achieve the desired reaction outcome through optimization, lowering the cost and time associated with catalyst development. After the initialization and optimization campaign, the trained model can be investigated for fundamental knowledge of the effects of process variables on reaction outcome.
Flow chemistry is another powerful tool that can reduce the time and cost associated with the discovery and development of chemical processes. Automated flow reactors can provide superior control over the reaction conditions, minimize human error, intensify transport rates, and improve process consistency. Furthermore, automation can be coupled to in-line analysis for monitoring and characterization of the reaction in real-time, providing feedback to adjust the reaction conditions and optimize the reaction process in a closed-loop format.
In this work, we extend our recent efforts of reaction condition optimization in a self-driving flow reactor to include discrete variables such as ligand structure as well as continuous process parameters. Extending the scope of the optimization to include ligand structure allows for the identification of an optimal ligand species given a ligand library and hydroformylation reaction target. The automated reactions in parallel batch and flow are leveraged alongside the ML-based experimental selection to perform autonomous regioselectivity tuning and optimization as well as developing a molecular structure-informed digital twin of the reaction.
Michael Bergman
mbergma2@ncsu.edu
Faculty Sponsor: Carol K. Hall
Project Title: Computational Discovery of Plastic-Binding Peptides for Microplastic Pollution Remediation
Project Abstract
Computational Discovery of Plastic-Binding Peptides for Microplastic Pollution Remediation
Micro- and nanoplastics (MNPs), defined as plastics on the micrometer or nanometer scale, are a concerning pollutant. Millions of tons of MNPs pervade the environment. This results in humans and other organisms unintentionally consuming MNPs, which can negatively impact health. It thus is urgent to develop methods for remediating MNP pollution. Noting that polypeptides can adsorb strongly to polymeric materials, we aim to computationally discover plastic-binding polypeptides (PBPs) to help detect, capture, and/or biodegrade MNP pollution. We focus in particular on the plastics polyethylene, polystyrene, polypropylene, and PET, which are common components of MNP pollution. To discover PBPs, we first used Peptide Binding Design (PepBD), a peptide design program based on biophysical modeling. PepBD sampled 105-106 peptides per plastic to identify a set of potential PBPs for each plastic. We next searched for additional PBPs by training a machine learning (ML) model on PepBD data. The ML model had two components: one that predicts peptide affinity (i.e., a PepBD score) based on the peptide’s amino acid sequence, and a second that optimizes the amino acid sequence with guidance from the score predictor. Specifically, the score predictor was LSTM while the sequence generator was Monte Carlo Tree Search. The tunability of the MCTS reward function allowed us to simultaneously optimize multiple peptide properties, giving rise to PBPs that also have high predicted solubility in water and bind with greater specifically to a target than to a non-target plastic. Measurement of peptide affinity in both molecular dynamics simulations and atomic force microscopy shows that both PepBD and ML PBPs have higher affinity to plastic than random peptides, and that ML designs have greater affinity to plastic than PepBD designs. We thus believe that the discovered PBPs can help develop bio-based methods that address MNP pollution.
Sina Jamalzadegan
sjamalz@ncsu.edu
Faculty Sponsor: Qingshan Wei
Project Title: Smart Agriculture: Early Detection of Plant Diseases Using Wearable Sensor Data and Machine Learning
Project Abstract
Smart Agriculture: Early Detection of Plant Diseases Using Wearable Sensor Data and Machine Learning
The United Nations predicts that by 2050, the global population will reach 10 billion, necessitating a 60% increase in food productivity to ensure food security. One of the primary threats to this goal is plant diseases, which result in crop losses ranging from 20 to 40% annually. These losses not only diminish food production but also have far-reaching consequences, including impacts on biodiversity, socioeconomic stability, and the cost of disease control, which together undermine global food security.
To address these challenges, innovative technologies are being developed within the realm of smart agriculture, such as wearable plant sensors. Our research focuses on a novel multimodal wearable sensor attached to the lower surface of plant leaves. This sensor continuously monitors plant health by tracking both biochemical and biophysical signals, as well as environmental conditions. The sensor platform integrates the detection of volatile organic compounds (VOCs), temperature, and humidity—critical indicators of plant stress and disease.
In addition to the wearable sensor technology, we have developed an unsupervised machine learning (ML) model using principal component analysis (PCA) designed to analyze the multichannel real-time data collected by the sensors. This model has shown promising results in the early detection of plant diseases, particularly the tomato spotted wilt virus (TSWV), which it can identify as early as four days post-inoculation. Furthermore, our analysis reveals that the accurate detection of this virus requires a combination of at least three sensors, with VOC sensors being crucial for reliable predictions.
This integration of advanced wearable sensor technologies with sophisticated machine learning techniques represents a significant step forward in smart agriculture. By enabling early detection of plant diseases, this system offers the potential to mitigate crop losses, reduce the costs associated with disease management, and contribute to more sustainable global food systems. Through innovations like these, we aim to support the global push towards increased food productivity and secure a more resilient future for agriculture.
Moritz Woelk
mwoelk@ncsu.edu
Faculty Sponsor: Wentao Tang
Project Title: Data-driven State Observation by Kernel Canonical Correlation Analysis
Project Abstract
Data-driven State Observation by Kernel Canonical Correlation Analysis
The difficult nature of modeling the states of chemical processes stems from the intrinsic nonlinearity within system dynamics. Deriving models that accurately represent the internal states of nonlinear processes is challenging due to the complexity of the governing equations, making the process both time-consuming and computationally expensive. In order to derive and reduce governing equations, knowing the system’s internal states is a prerequisite. Therefore, constructing a state observer, specifically an auxiliary dynamical system that uses the system’s inputs and outputs to estimate the internal states, allows for the inference of the system’s behavior without requiring direct measurements of those internal states. By solely utilizing accessible system data, the states’ information can be determined by finding the relationship between past and future input and output measurements.
Thus, kernel canonical correlation analysis (KCCA) is adopted in this work for the reconstruction of a state sequence from a nonlinear dynamical system that incorporates both past and future data, circumventing the need for physics-informed models. Specifically, by using a nonlinear kernel function, which maps the data into a feature space, we employ canonical correlation analysis (CCA) to find a subspace from two datasets (i.e., past and future input and output measurements) with maximal correlation. Based on the formulation using Mercer’s Theorem and the kernel trick, an appropriate form of regularization can be further added by adopting a least squares support vector machine (LS-SVM) approach with KCCA. In this formulation, the squared error is minimized with regularization on the weight vectors. This regularized KCCA is a convex optimization problem, which results in solving a generalized eigenvalue problem in the dual space. This approach is amenable to artificial intelligence (AI) through the utilization of machine learning techniques (such as kernel methods and support vector machines) to learn a reconstruction of the states by finding relationships between two datasets, resulting in a representation that closely matches the true states. The application to a numerical example and exothermic continuously stirred reactor (CSTR) case study demonstrates that KCCA is a sufficient, performance-guaranteed, model-free state observer, provided adequate data.
Jinge Xu
jxu49@ncsu.edu
Faculty Sponsor: Milad Abolhasani
Project Title: Smart Manufacturing of Metal Halide Perovskite Nanocrystals Enabled by a Multi-Robot Self-Driving Lab
Project Abstract
Smart Manufacturing of Metal Halide Perovskite Nanocrystals Enabled by a Multi-Robot Self-Driving Lab
All-inorganic Metal halide perovskite (MHP) quantum dots (QDs) have emerged as a highly promising class of semiconducting nanomaterials for various solution-processed photonic devices. These quantum-confined nanocrystals exhibit unique optical properties that can be precisely engineered by altering their composition, shape, size, and geometry(1). The surface ligation of MHP QDs relies on an acid-base equilibrium reaction, which is commonly utilized not only to provide colloidal stability in organic solvents but also to tune their optical properties. The use of different organic acids as surface ligands leads to distinct growth pathways and thereby different QD morphologies(2). Consequently, the optical characteristics of MHP QDs are strongly affected by both the ligand structure (discrete parameter) and the precursor concentrations (continuous parameters). The multidimensional nature of this parameter space makes it extremely challenging to efficiently explore and understand the underlying mechanism of synthesis. Traditional synthesis methods for MHP QDs are time-consuming, material-intensive, and laborious, relying on manual flask-based techniques. The manual nature of these methods, along with the interdependent reaction and processing parameters in colloidal QD synthesis, hinders the discovery of optimal formulations and fundamental understanding of MHP QDs(3).
In this work, we have developed a multi-robot self-driving lab (SDL) for accelerated synthesis space mapping of room temperature-synthesized colloidal semiconductor nanocrystals. The developed SDL enables automated and closed-loop investigation of the effects of ligand structure and precursor concentrations on the photon-conversion efficiency, nanocrystal size uniformity, and bandgaps of MHP QDs. Next, we utilized the developed multi-robot SDL to autonomously map the pareto-front of MHP QDs’ optical properties for multiple target peak emission wavelengths.
We overcame challenges of conventional applied and fundamental QD research by investigating the science and engineering of a modular intelligent robotic experimentation platform. We established a closed-loop QD synthesis and development strategy by integrating a modular robotic experimentation platform with data-driven modeling and experiment-selection algorithms. The developed SDL accelerated mapping the optical properties of MHP QDs to the ligand structures and synthesis conditions and understanding the underlying role of ligand structure on the shape, morphology, and optical properties of MHP QDs. The SDL-generated knowledge will enable on-demand synthesis of MHP QDs with optimal optical properties for the next generation energy and display technologies.
Civil, Construction and Environmental Engineering
Ange Therese Akono
aakono@ncsu.edu
Faculty Sponsor: Ange Therese Akono
Project Title: Novel recycled aggregate concrete using nanomaterials and AI
Project Abstract
Novel recycled aggregate concrete using nanomaterials and AI
The use of recycled concrete aggregates for new concrete production is an important avenue to increase the sustainability of concrete. While numerous studies have demonstrated the benefit of nanosilica on recycled aggregate concrete, a connection between the enhanced properties and the properties of the calcium-silicate-hydrates is unproven. Thus, using AI, we investigate how nanosilica modifies the distribution of calcium-silicate-hydrates in fine recycled concrete aggregate mortar. Using an interdisciplinary approach of nanomechanical testing, data science, and advanced chemical, microstructural and pore structure characterization, we find that 12 wt.% nanosilica results in a 30.8% increase in the combined relative amount of high-density and ultra-high-density C-S-H, 16.7% reduction in C-S-H gel porosity, 24.4% reduction in total porosity, and 3.9% increase in fracture toughness.
Kichul Bae
kbae2@ncsu.edu
Faculty Sponsor: Sankar Arumugam, Ranji Ranjithan
Project Title: AI-based Flood Prediction: Role of ML in Water Depth and Flow Estimation from Camera Images
Project Abstract
AI-based Flood Prediction: Role of ML in Water Depth and Flow Estimation from Camera Images
Early warning of flooding, which helps minimize loss of life and property, requires integration of different physics-based models (e.g., atmospheric models, watershed models and urban stormwater models) and current water level and streamflow conditions to forecast spatio-temporal variations in flooding. Combining the quantitative model predictions with site-specific experiential knowledge from past flood events enables an artificial intelligence (AI)-based flood prediction framework to provide targeted flood warnings for the community. A major challenge is finding water level and streamflow data at local scale to capture community-level flood inundation. Stream gauges, which are sparsely distributed, provide data only at the river basin scale that are inadequate to capture flood inundation especially in urban areas. Fortunately, ubiquitous video images are available (e.g., from cameras used for traffic monitoring, public surveillance and home security) as potential sources for extracting water level data. We are developing a machine learning (ML) approach, using convolution neural networks, to first associate the inundation features in the images with the limited stream water level and flow data. These ML-models utilize city-wide camera images to estimate water level and flow at an urban extent. This data can also be used to calibrate and validate the physics-based watershed and stormwater models to correct systematic errors associated with physics-based models and enhance the flood warnings for locations with limited flood data. Initial investigations based on thirteen locations with USGS stream gauges in Mecklenburg county in NC show potential in utilizing camera images in the proposed approach to issue city-wide flood warnings.
Lochan Basnet
lbasnet@ncsu.edu
Faculty Sponsor: Kumar Mahinthakumar, Ranji Ranjithan
Project Title: Role of Machine Learning Approaches in Localizing Pipe Leaks in Water Distribution Systems (WDSs)Distribution Systems
Project Abstract
Role of Machine Learning Approaches in Localizing Pipe Leaks in Water Distribution Systems (WDSs)
Leak localization is critically important for water utilities worldwide, as the significant loss of treated water each year results in substantial economic, infrastructural, and environmental impacts. However, addressing leak localization remains challenging due to the prohibitive costs of physical solutions and the limitations associated with data-driven, model-based approaches, exacerbated by limited data availability. While the simulation of hydraulic models has mitigated data limitations, further advancements are required in both the conceptualization and formulation of the leak localization problem, as well as in algorithmic development. A review of the current state of research reveals that most model-based studies, although promising, rely on simplifying assumptions that do not adequately capture the complexities of real-world leak localization in water distribution systems (WDSs). These assumptions hinder the practical application of these methodologies in addressing actual leaks.
Our research aims to provide a data-driven leak localization solution that holistically examines the leak complexities, focusing on various critical aspects such as the expansiveness of WDSs, leak characteristics, uncertainties, leak characterization resolution, and the integration of multi-input data. At the core of our approach are machine learning (ML) algorithms, which have demonstrated their efficacy across numerous domains, including WDSs. Considering the wide array of ML algorithms available, our selection of three established ML models—Multilayer Perceptron (MLP), Convolutional Neural Networks (CNN), and Transformers—offer an adequate space for exploration and comparison. Our ML-based approach for comprehensive leak localization was tested on a large-scale WDS in a major metropolitan area of California under both simulated and real-world leak scenarios. The results are highly promising, with high accuracy achieved in both simulated and real settings.
Gongfan Chen
gchen24@ncsu.edu
Faculty Sponsor: Edward Jaselskis
Project Title: Meet2Mitigate: An LLM-Powered Framework for Real-Time Issue Identification and Mitigation from Construction Meeting Discourse
Project Abstract
Meet2Mitigate: An LLM-Powered Framework for Real-Time Issue Identification and Mitigation from Construction Meeting Discourse
Construction meetings are essential for bringing together project participants to coordinate efforts, identify problems, and make decisions. Previous studies on meeting analysis relied on manual approaches to identify isolated pieces of information but struggled with providing a high-level overview that targeted real-time problem identification and resolution. Despite the rich discussions that occur, the sheer volume of information exchanged can make it difficult to discern key issues, decisions, and action items. Recent advancements in large language models (LLMs) provide sophisticated natural language processing capabilities that can effectively distill essential information and highlight actionable insights from meeting transcripts. However, these technologies are often underutilized in practice, despite their potential to significantly enhance the analysis and management of meeting data. This research introduced the Meet2Mitigate (M2M) framework, which integrates cutting-edge technologies, including speaker diarization, automatic speech recognition (ASR), LLMs, and retrieval-augmented generation (RAG) to revolutionize how construction meetings are captured and analyzed. In this framework, construction meeting recordings can be converted into a structured format, differentiated by timestamps, speakers, and corresponding contents. Different speakers’ dialogues are then summarized to extract the main project-related issues. For quick mitigation responses, this framework combines LLMs with a retrieval mechanism to access the Construction Industry Institute (CII) Best Practices (BPs) knowledge pool, generating detailed action items to drive problem solving. The validation results demonstrated that the M2M prototype can automatically generate a tailored end-to-end problem-to-solution report in real-time by only using a meeting recording file. The resulting problem-to-solution reports can be promptly shared with project teams, including non-attendees, ensuring an effective meeting recap and quick alignment on decisions among the teams.
Panji Darma
pndarma@ncsu.edu
Faculty Sponsor: Moe Pourghaz
Project Title: Imaging Biological Healing in Concrete Vasculature Network Using Hybrid Electromagnetic Method and Convolutional Neural Network
Project Abstract
Imaging Biological Healing in Concrete Vasculature Network Using Hybrid Electromagnetic Method and Convolutional Neural Network
Microbially Induced Calcium Carbonate Precipitation (MICP) is an innovative bio-based technique that has gained significant attention for its potential to enhance the healing and durability of concrete. This process utilizes specific bacteria, such as S. Pasteurii, which precipitate calcium carbonate (CaCO₃) as a byproduct of their metabolic activities, filling cracks and improving structural integrity. However, the efficiency and effectiveness of MICP rely on a comprehensive understanding of the spatial and temporal dynamics of CaCO₃ precipitation within the concrete matrix. To monitor the MICP process, we propose a novel imaging approach that combines Ground-Penetrating Radar (GPR) and Electrical Impedance Tomography (EIT), enhanced by Convolutional Neural Networks (CNN). GPR is a well-established method for detecting subsurface cracks in concrete through reflection waves, while EIT effectively visualizes biological activity via conductivity changes. By integrating these imaging techniques with CNN, we aim to achieve high-resolution, real-time visualization of the biological healing process within concrete vasculature networks. Our approach combines these techniques with CNN in a three-stage process: (a) identifying subsurface cracks using GPR reflection waves and training the CNN to generate a 3D geometry of the cracks; (b) performing planar EIT finite element computation based on the subsurface crack geometry provided by the CNN; and (c) monitoring the MICP process using planar EIT. This approach promises to improve the monitoring, optimization, and overall effectiveness of MICP in concrete, paving the way for more durable and sustainable construction materials.
Shiqi Fang
sfang6@ncsu.edu
Faculty Sponsor: Sankar Arumugam
Project Title: A Complete Density Correction of GCM Projections Using Normalizing Flows
Project Abstract
A Complete Density Correction of GCM Projections Using Normalizing Flows
Global Climate Models (GCMs) provide essential information for impact analysis, adaptation pathways, and prediction studies. However, GCMs often contain systematic errors that require correction against observations before their use in impact assessments, especially for application studies. Traditional univariate bias corrections based on quantile mapping do not preserve cross-relations across multiple variables. Despite recent advances in multivariate bias correction methods which perform better than univariate methods, fully correcting the joint density of multiple variables from GCM projections still remains challenging. In this study, we propose an AI-based density correction method, normalizing flow (NF), to correct precipitation (P) and near-surface maximum air temperature (T) from CMIP6 projections. NF offers several advantages over traditional methods, including the ability to model complex, multidimensional dependencies and transform the distribution of variables to match the joint probability density function (PDF) of observed data. We assess the performance of NF-corrected CMIP6 projections over the historical period (1951–2014) across the continental US, focusing on the ability to reproduce inter-variable relationships and spatio-temporal consistency. Results indicate that NF effectively corrects biases in the joint density of CMIP6 projections and also preserves spatial patterns and cross-correlation between variables. Compared to other methods such as MACA, quantile mapping, and canonical-correlation analysis, NF best matches the PDF of observations with the smallest Wasserstein distance both spatially and temporally for P and T, and better quantifies extreme events compared with observations. NF also demonstrates consistently strong performance across different seasons and climate regions. Thus, NF could not only bias-correct moments in observed variables but also correct the joint density of climate variables from CMIP6 projections, thereby showing promise in improving the estimation of hydroclimatic extremes using GCM projections.
Krishna Mohan Ganta
gkrishn5@ncsu.edu
Faculty Sponsor: Jacqueline MacDonald Gibson
Project Title: ASSESSING PERFORMANCE OF A HYBRID MECHANISTIC GROUNDWATER / MACHINE-LEARNED BAYESIAN NETWORK (MLBN) MODEL
Project Abstract
ASSESSING PERFORMANCE OF A HYBRID MECHANISTIC GROUNDWATER / MACHINE-LEARNED BAYESIAN NETWORK (MLBN) MODEL
To better manage emerging public health challenges, communities, water utilities, and regulators must improve methods to assess and prioritize environmental health risks. PFAS exposure in North Carolina is a prime example where methodologies for predicting and mitigating risks are developing concurrently with contaminant regulations and the allocation of mitigation funding. This project aims to predict the risk of private wells exceeding the provisional health goal for the PFAS GenX. PFAS compounds are notoriously difficult to model with mechanistic groundwater flow and fate and transport models. The sheer number of different PFAS chemicals and uncertainty in their individual and interacting characteristics, all make them complex to model in the environment. Mechanistic models are also resource-intensive to develop and calibrate. This project builds upon previous work that developed a Machine-Learned Bayesian Network (MLBN) classification model to predict at-risk wells; current work integrates outputs from a mechanistic groundwater fate and transport model as input variables to new MLBNs, classified as low-, medium-, and high-effort models in terms of mechanistic modeling resources required. Performance of each model is compared to the mechanistic model predictions of at-risk wells using several performance metrics, including accuracy, area under the receiver operating characteristic curve (AU-ROC) and F-score curves, and the importance of each metric and model performance is discussed in the context of environmental health risks. Results show that MLBNs perform as well as the mechanistic models in accuracy and AU-ROC performance metrics, while being more robust in terms of the range of decision thresholds selected for risk classification. High-effort models make slight improvements in AU-ROC metrics, while more easily incorporating insights from mechanistic model performance without the need to recalibrate the mechanistic model. The project aims to assist regulators to advance public health and methodologies to integrate traditional engineering models with machine-learning approaches.
Timothy Leung
tleung@ncsu.edu
Faculty Sponsor: Jacqueline MacDonald Gibson
Project Title: Targeting Homes with High Lead Exposure Risks by Leveraging Big Data and Advanced Machine-Learning Algorithms
Project Abstract
Targeting Homes with High Lead Exposure Risks by Leveraging Big Data and Advanced Machine-Learning Algorithms
Although improvements to lead exposure have been quite significant in recent decades, there is a current misconception that lead exposure is no longer a health issue due to its elimination from many products. The World Health Organization still identifies lead as one of the top 10 chemicals of public health concern among workers, children, and women of reproductive age as of 2023. While the prevalence of elevated blood lead levels is gradually declining, it has been estimated that every 1 in 3 children is not screened and reported for elevated blood lead at both the state and federal levels. Even among adults, the accumulation of lead exposures from the home and work environment can lead to reduced heart, brain, and kidney functions down the road.
Past and existing efforts to mitigate or eliminate household lead exposures still face several challenges. Currently, Pb remediation in the U.S. is often triggered by the detection of elevated Pb in a child’s blood. Relying on children as sentinels of Pb exposure risk means that remediation may come too late, after the damage is done. In addition, it fails to prevent lower levels of exposure, which may not increase blood Pb enough to require an inspection but may nonetheless increase the risk of cognitive damage. Due to the complexity of multi-media lead exposure routes, it is difficult to identify and prioritize areas within the home that may have varying levels of lead. Hazard identification approaches based on simple thresholds of house age and/or census-level demographic information fail to capture opportunities that arise from integrating multiple data sources. When combined systematically, integrated data sources can reveal important interactions among risk factors that may have synergistic effects.
This project applies a precision healthy housing approach where statistical and machine learning models are trained to better predict which houses are at risk and which interventions are likely to be most effective against these context-specific hazards. Results of preliminary models trained on big datasets curated from tax parcel, demographic, and physical/environmental data sources in NC, demonstrate a marked improvement in true positive rate (50 to 80%) over existing lead risk criteria in identifying individual homes for remediation, with fewer resources wasted on false positives, and without relying on children’s BLL to trigger remediation after exposure. BLL test year, year of home construction, median census block group household income, percent of block group identifying as Black, number of drinking water system connections, and child age are some of the most influential variables in predicting lead risk. Next steps are to identify the optimal model performance, subset of input variables required, risk threshold, and data resolution required to achieve these risk prediction improvements.
Jessica Levey
jrlevey@ncsu.edu
Faculty Sponsor: Sankar Arumugam
Project Title: Subseasonal-to-Seasonal Streamflow Forecast
Project Abstract
Subseasonal-to-Seasonal Streamflow Forecast
Climate change is altering global hydroclimatology and threatening the majority of the world’s fresh water sources. In addition to climate change, population and land use change are stressing water sources and will amplify the water scarcity crisis. Rivers are dammed to create reservoirs, which serve various purposes including water supply, flood control, hydropower, and irrigation. Reservoir releases are modified during droughts and floods to meet water demand without increasing downstream risk. Mismanagement of water resources may threaten agriculture and food supply chains, human and environmental health, and regional economies. Accurate streamflow forecasts is essential for effective water resource management and planning. Most reservoirs are operated by only considering short-range inflow forecasts, 1-7 days lead time, which is important for determining daily releases to meet the daily water supply and energy demands and as for managing flash flood and storm-caused flood events. Reservoir operations do not consider forecasts at the subseasonal-to-seasonal (S2S) range, 15-90 days ahead. S2S forecasts are important energy grid planning, meeting storage demands for irrigated agriculture, drought effect mitigation, and maintaining long term storage and flow conditions for meeting ecological demands. This study a) develops a large-scale framework using a multivariate long short term memory (LSTM) model t0 predict S2S streamflow forecasts for large-scale water resource management and b) evaluates the forecast performance for below normal, normal, and above normal flows. As hydrologic extremes are becoming more frequent and intense, accurate, longer-range forecasts will be crucial for meeting water demand and effectively managing water resources.
Harleen Kaur Sandhu
hksandhu@ncsu.edu
Faculty Sponsor: Abhinav Gupta
Project Title: Digital Twin for Nuclear Piping Systems: Harnessing Deep Learning for Advanced Condition Monitoring
Project Abstract
Digital Twin for Nuclear Piping Systems: Harnessing Deep Learning for Advanced Condition Monitoring
Advanced nuclear reactors represent a significant breakthrough in energy technology due to their compact design, enhanced robustness, superior reliability, and high capacity factors. These reactors are particularly suitable for deployment in remote areas or regions affected by disasters, providing a critical and dependable power supply in challenging conditions. Their high efficiency and reduced greenhouse gas emissions make them a viable choice for sustainable energy production. To facilitate widespread adoption, it is important to minimize life-cycle maintenance costs while ensuring the long-term structural integrity of the reactors.
Recent advancements in artificial intelligence (AI) and deep learning algorithms have made Digital Twin (DT) technology a practical solution for the autonomous operation of advanced nuclear reactors. Enhanced DT frameworks present a cost-effective solution for the continuous monitoring of system health, particularly in safety-critical components such as piping and equipment systems. These systems play a vital role in the efficient transfer of coolant within nuclear facilities. Yet, they are susceptible to flow-accelerated corrosion and erosion, which can cause thinning of pipe walls and, if unchecked, lead to critical infrastructure failures.
Traditional non-destructive testing (NDT) methods, while effective, are labor-intensive and often necessitate the shutdown of power plants, resulting in substantial financial impacts on electric utility companies. The integration of AI-driven DTs with advanced condition monitoring capabilities allows for real-time analysis of sensor data, providing early warnings of degradation and enabling proactive maintenance. Machine learning algorithms, particularly those trained on large datasets, are adept at detecting subtle signs of structural damage in piping systems, thereby reducing reliance on costly NDT equipment and decreasing the frequency of plant outages required for inspections.
This research proposes a diagnostic digital twin for nuclear piping systems, including their design, construction, and continuous condition monitoring throughout their operational life. During the design and construction phases, digital tools are developed to facilitate the seamless integration between Building Information Modeling (BIM) software and Finite Element (FE) structural design software, ensuring accurate virtual compatibility checks and proper installation fit. The condition monitoring framework leverages AI-based methodologies to detect and quantify degradation, such as pipe wall thinning due to flow-accelerated corrosion (FAC), which occurs as a result of the transportation of high-temperature and high-pressure fluids in nuclear power plants.
A laboratory-based demonstration of an “as-built” diagnostic digital twin is conducted, focusing on a piping system subjected to conditions representative of real-world nuclear facilities. This demonstration involves both simulated and empirical data to track aging and degradation over time. The case study includes a laboratory installation where the piping system undergoes controlled vibrations to develop a robust diagnostic workflow. Deep learning algorithms, including Multi-Layer Perceptrons (MLPs) and Convolutional Neural Networks (CNNs), are evaluated for their diagnostic accuracy and computational efficiency. Additionally, advanced data processing techniques, such as domain randomization, are employed to enhance the generalization and reliability of the AI models. By integrating AI with DT technology, this research aims to aid the maintenance and operation of advanced nuclear reactors, ensuring their safety, efficiency, and longevity.
Liannian Wang
lwang64@ncsu.edu
Faculty Sponsor: Kevin Han, Abhinav Gupta
Project Title: Advancing Construction Requirements Management through Generative AI-Driven Workflow
Project Abstract
Advancing Construction Requirements Management through Generative AI-Driven Workflow
“Construction engineering, particularly for large-scale projects like nuclear power plants, is inherently complex and requires strict adherence to high-quality and safety standards. Ensuring that construction activities align with approved designs and standards is crucial for safeguarding the facility, the environment, and human life. However, 98% of megaprojects experience an average cost overrun of 80% and a delay of 20 months, with ineffective management of construction requirements being a critical factor. The current documentation-based manual processes are labor-intensive, time-consuming, and prone to errors and omissions, leading to significant vulnerability in cost overruns and delays. While advanced technologies exist, they are often unsuitable or difficult to apply to most construction projects, requiring further optimization. These challenges highlight the urgent need for innovative solutions incorporating advanced technologies, particularly artificial intelligence (AI), to streamline processes and democratize intelligence in construction projects.
This research proposes a transformative approach to construction requirements management by integrating generative AI (GenAI) into the workflow. The goal is to shift from manual and error-prone practices to data-driven automated systems, thereby enhancing the efficiency and accuracy of managing construction requirements. GenAI, with its streamlined model-building and training process, offers an accessible and cost-effective alternative to traditional construction requirements management, making intelligence more accessible to a broader range of users.
The GenAI integrated process begins by developing a well-structured and domain-specific ontology. This ontology is the foundation for the AI’s understanding of construction-specific terminology and relationships, providing a significant framework that ensures accurate, consistent, and contextually relevant outputs. By guiding the AI’s interpretation of domain-specific contexts, the ontology enables GenAI to deliver precise results that align with industry standards. Unlike other methods that require extensive domain expertise and technical knowledge, the ontology-supported GenAI approach is more adaptable and scalable. It allows for the seamless conversion of extensive requirement documents into structured inputs for the GenAI system, significantly speeding up the process and reducing the risk of errors typically associated with manual documentation practices. Then, to support this workflow, a user-friendly platform will be developed to facilitate collaboration among project stakeholders, ensuring smooth data transfer and interaction. Moreover, the GenAI capabilities will be extended to analyze project performance data, providing stakeholders with informed decision-making support through clear and accessible reporting mechanisms. This comprehensive workflow aims to enhance construction requirement management and project performance using advanced AI technology, fostering effective stakeholder collaboration, and delivering real-time insights to practitioners. By expediting decision-making and improving project outcomes, this approach envisions a future where AI-driven intelligence becomes a universal asset in the construction industry, making advanced technological support accessible and beneficial to all stakeholders involved.”
Computer Science
Muhammad Alahmadi
mjalahma@ncsu.edu
Faculty Sponsor: Dongkuan Xu
Project Title: Reliable Synthetic Data Generation
Project Abstract
Reliable Synthetic Data Generation
The emergence of Large Language Models (LLMs) represents a major advancement in Artificial Intelligence (AI). These models can generate synthetic data, which plays a crucial role in boosting LLM performance and facilitating the training of smaller models, particularly when high-quality datasets are limited or unavailable. One key factor in the effectiveness of synthetic data is diversity, which is essential for enhancing the robustness and generalization capabilities of the models. However, despite the significant role of data diversity in improving model performance, more comprehensive research needs to be conducted focused on optimizing methods for data diversification and developing evaluating measures of data diversity. This gap in research highlights the need for more in-depth studies to better understand and refine diversification techniques to improve synthetic data quality and model outcomes.
My research addresses the existing gap by critically evaluating current methods for data diversification and the metrics used to assess diversity in synthetic datasets. It examines the effectiveness of existing diversification strategies and proposes innovative approaches to advance data generation techniques. Key areas of exploration include the integration of Retrieval-Augmented Generation (RAG) and the investigation of the impact of seed corpus diversity and selection processes on synthetic data generation. Additionally, my research aims to incorporate methodologies and concepts from related fields, such as data distillation and active learning. These aspects are underexplored in the literature and represent promising avenues for enhancing the quality of synthetic data.
To accurately evaluate the effectiveness of diversification methods, it is essential to develop robust assessment techniques for data diversity. These techniques should extend beyond the commonly studied lexical diversity to include the semantic dimension, with particular emphasis on its relevance to the specific task at hand, which could result in varying semantic spaces depending on the context of the task and the data.
The domain of my research is in textual datasets and benchmarks which are used to assess language understanding capability of the model. Robustness, adaptability, and generalization of the models are important in building reliable and trustworthy AI models. Increased reliability will ensure consistent performance across diverse tasks, while advancements in self-awareness and self-improvement mechanisms may facilitate greater model autonomy and adaptability.
By addressing the limitations of current synthetic data generation methods and emphasizing data diversity, my research seeks to develop more capable and generalizable AI models. Such improvements are vital for overcoming challenges related to data scarcity and quality, thus advancing toward the realization of Artificial General Intelligence (AGI), where AI systems exhibit the capability to perform a wide array of tasks, continuously learn, and autonomously evolve.
Adrian Chan
amchan@ncsu.edu
Faculty Sponsor: Chau-Wai Wong
Project Title: Handwritten Text Recognition (HTR) for Historical Arabic Documents
Project Abstract
Handwritten Text Recognition (HTR) for Historical Arabic Documents
Handwritten text recognition (HTR) involves taking an image that contains handwritten text and converting it into the corresponding characters on a computer. HTR is a longstanding challenge in computer vision and machine learning due to the significant variability in handwriting styles among individuals. Our project focuses on HTR for Arabic handwriting, which comes with additional challenges compared to HTR for English handwriting. Arabic handwriting is written in cursive, context-sensitive, and features connected characters, complex ligatures, and diacritics. Furthermore, the availability of Arabic handwriting datasets is limited compared to English datasets, further increasing the difficulty of training generalizable HTR models. This work proposes a transformer-based neural network to address the unique challenges of transcribing historical Arabic handwriting. We show that image and text preprocessing modifications, synthetic datasets, model pretraining, and overtraining can help adapt state-of-the-art English HTR models to Arabic. We evaluated our proposed model with other Arabic recognition methods using character error rate (CER). Experiments show that our proposed HTR method outperforms all other methods with a CER of 8.6% on the largest publicly available handwritten Arabic text curated by our team.
LiChia Chang
lchang2@ncsu.edu
Faculty Sponsor: Dongkuan (DK) Xu
Project Title: Diagnostic Chatbot for SAP/APM Manufacturing Based on Agentic LLM: A Real-World Industry Application
Project Abstract
Diagnostic Chatbot for SAP/APM Manufacturing Based on Agentic LLM: A Real-World Industry Application
Large Language Models (LLMs) have rapidly gained prominence across various fields, including automated manufacturing domains. In the context of SAP (Systems, Applications, and Products) and Application Performance Management (APM), which are widely used tools that help businesses manage finance, logistics, and supply chain, LLMs offer significant potential. These systems manage terabytes of numeric and textual log data generated from by sensors and human operators within manufacturing environments. Traditionally, diagnosing issues from such data has required the expertise of several data scientists and domain specialists with strong background knowledge, who manually analyze each problem. Although some machine-learning techniques have been developed to analyze such data, several limitations persist. For example, the results generated by these techniques often require interpretation by analysts, as deriving meaningful insights from the data can be challenging without deep domain knowledge. This reliance on expert interpretation can hinder the efficiency and accessibility of automated data analysis and problem shooting in manufacturing environments. Moreover, traditional natural language processing techniques lack a comprehensive solution for handling massive textual data. These techniques are often limited to basic keywords extraction and string matching, which are insufficient for capturing the full complexity of textual messages in industrial contexts.
To harness the capability of LLM-empowered tools, we developed a free-form question-answering chatbot built on an Agentic LLM system, utilizing Synergizing Reasoning and Acting (ReAct) criteria. This system is integrated with custom-defined tools to address the nature of APM/SAP data and a wide range of inquiries from workers in the manufacturing environment. Concretely, user inquiries can be categorized into four types: questions that do not require any contextual information, questions that necessitates database retrieval, questions that require semantic search, and malicious queries aimed at obtaining protected information through prompt injection attacks. To address these types of inquiries, the Agentic LLM is integrated with specialized tools, each tailored to solve a specific category of question. These tools include: a LLM-based SQL translator which operates with the full SAP/APM database schema provided in the system prompt, a SQL engine that executes queries and returns the retrieved results, Retrieval-Augmented Generation (RAG) for semantic search, and a LLM-based final checker to ensure that the final response does not contain unrelated or confidential content. All credit for this work goes to PrometheuseGroup, Headquarters – Raleigh, NC.
Teddy Chen
xchen87@ncsu.edu
Faculty Sponsor: Shiyan Jian, Dongkuan Xu
Project Title: Unveiling Implicit Bias in LLM-Generated Educational Materials: A Prompt-Based Approach with BiasViz
Project Abstract
Unveiling Implicit Bias in LLM-Generated Educational Materials: A Prompt-Based Approach with BiasViz
Large Language Models (LLMs) are increasingly utilized in educational settings for tasks like personalized learning, content generation, and assessment. However, these models often perpetuate implicit biases rooted in their training data, including gender, geographic, and social biases, which can subtly influence educational outcomes. Traditional bias detection methods focus on explicit biases, often relying on predefined lists of stereotypes. However, implicit biases—those that operate unconsciously—remain a significant challenge and can lead to unequal learning experiences. This project proposes a novel prompt-based approach to uncover and measure implicit bias in LLM-generated educational content. We will design a dataset of stereotype-laden prompts, subtly embedding information through names, contexts, and cultural references, and use it to evaluate widely-used LLMs like GPT-4 and LLaMA. By analyzing LLM outputs for bias in scenarios such as writing assistance and grading, we aim to provide a more comprehensive understanding of how these models
function and where they may falter.
To support our research, we have designed and developed BiasViz, an innovative interactivetool that allows users to visualize, analyze, and mitigate biases in LLM-generated content.Initially developed as a prototype focused on gender bias, BiasViz can be easily expanded tostudy broader bias scenarios, including geographic and social biases. The prototype hasalready been deployed at BiasViz (https://llm-biasviz.teddysc.me/), enabling studentsand researchers to explore biases through dynamic visualizations, such as next-wordprobability and biased phrase highlighting, making the often opaque nature of LLMs moretransparent. The tool also allows users to create counter-narratives and dynamically linksdetected biases to their sources within the training data. Additionally, BiasViz providesfunctionality for bias quantification and mitigation strategies, drawing on frameworks likeIBM AI Fairness 360. By incorporating BiasViz into our research, we aim to empower users to
actively participate in the process of bias identification and correction, contributing to the development of fairer and more inclusive educational AI systems. This project is guided by Dr. DK Xu from the Department of Computer Science at NC State, Dr. Shiyan Jiang from the College of Education at NC State, and Dr. Duri Long from the School of Communication at Northwestern University, all experts in AI fairness, educational technology, and human-centered computing.
Aldo Dagnino
adagnin@ncsu.edu
Faculty Sponsor: Aldo Dagnino
Project Title: Condition Monitoring of Hydro-power Turbines in IIOT Power Generation Plants
Project Abstract
Condition Monitoring of Hydro-power Turbines in IIOT Power Generation Plants
This poster provides a framework used to conduct advanced analytics in an Industrial Internet of Things (IIoT) ecosystem for condition monitoring of smart networked and instrumented rotating pieces of equipment in power generation plants. The analysis of data that originates from an instrumented hydro-power generation turbine is used to demonstrate the capabilities of the analytics approach. A discussion of the main components that make a rotating machine “smart and networked” is provided. As data analytics is an essential component of an IIoT system, a discussion on different analytic approaches to analyze sensor data to monitor smart, instrumented, and networked rotating machines is discussed. These approaches are based on Machine Learning algorithms that can be used to identify patterns and to identify potential anomalies on a rotating piece of equipment. The analytic approaches derive knowledge through calculation of key performance indicators (KPIs) that are derived from analyzing sensor data from instrumented rotating pieces of equipment utilizing analytical methods such as statistical models, unsupervised clustering algorithms, and anomaly detection and projection algorithms. A presentation is given on how the KPI’s generated by the analytic methods translate into actionable knowledge used by a Service Engineer domain expert to address any anomalies in the rotating piece of equipment being monitored. Finally, a discussion on how a smart, instrumented, and networked rotating machine operates within the IIoT ecosystem is provided.
Jonah Ghebremichael
jghebre@ncsu.edu
Faculty Sponsor: William Enck, Alexandros Kapravelos
Project Title: Integrating Static Analysis with LLMs
Project Abstract
Integrating Static Analysis with LLMs
Static Application Security Testing (SAST) tools are vital parts of modern software security providing automatic feedback on potential vulnerabilities that can impact codebases. Unfortunately, for languages such as Javascript and Python, which possess dynamic features (callbacks, anonymous functions, etc), SAST tools lack code context when generating intermediate representation for these languages, leading to high false positives. In this report, we present MiCaSa, a system that introduces context-awareness via large language models (LLM) into static analysis tools. MiCaSa improves the reachability of intermediate representations such as call graphs by integrating LLMs into static code analysis. We modified the open-source SAST tool, Joern, and integrated LLM calls into areas where Joern failed to identify function callees appropriately. Our results show that it is possible to utilize LLMs to resolve the dynamic features in modern programming languages that present challenges to SAST tools. We believe that this approach can be extended to other SAST tools like CodeQL to provide enhanced vulnerability detection to a large number of codebases.
Nikolaos Pantelaios
npantel@ncsu.edu
Faculty Sponsor: Alexandros Kapravelos
Project Title: Building an LLM Deobfuscator for JavaScript Code
Project Abstract
Building an LLM Deobfuscator for JavaScript Code
JavaScript obfuscation is a widely used technique by both malicious actors and legitimate developers to conceal the intent and functionality of their code. Over 25% of malicious JavaScript is obfuscated, posing significant challenges in cybersecurity. This research presents an innovative approach to deobfuscating JavaScript code using Large Language Models (LLMs) with open-source weights, offering a novel solution to enhance code analysis and threat detection capabilities.
Our study addresses key research questions, such as identifying the categories of obfuscation techniques that can be effectively deobfuscated by our tool, evaluating the percentage of code that maintains functionality post-deobfuscation, and exploring the model’s capability to generate meta-output commands for user-guided deobfuscation when direct deobfuscation fails.
We utilize state-of-the-art LLMs, including Meta-Llama-3.1-70B-Instruct, mistralai/Mistral-Large-Instruct-2407 (123B), and deepseek-ai/DeepSeek-Coder-V2-Instruct-0724 (236B), on a comprehensive dataset of 100,000 pairs of obfuscated and deobfuscated JavaScript snippets. To ensure robustness, we generate diverse obfuscated samples using tools like obfuscator-js, UglifyJS, and JScrambler, covering techniques such as variable and function renaming, string encoding, control flow flattening, and dead code insertion. To address the challenge of hallucinations—generating incorrect code—we incorporate Retrieval-Augmented Generation (RAG) models, which leverage external knowledge sources for contextually accurate deobfuscation outputs.
The effectiveness of our LLM-based deobfuscator is evaluated using a range of NLP-based metrics, including BLEU Score, Edit Distance, METEOR, ROUGE-L, BERT-based Semantic Similarity, API Sequence Similarity, and AST Similarity. Additionally, dynamic execution metrics, such as the successful execution rate of deobfuscated code, provide further insights into the tool’s capabilities. Preliminary results indicate that larger models, such as Meta-Llama-3.1-70B-Instruct, demonstrate higher accuracy in producing readable and functional deobfuscated code, while smaller models may struggle with complex obfuscation patterns.
This research contributes to the field by providing an open-source, scalable, and efficient tool for deobfuscating JavaScript code, addressing a critical need for more effective defenses against obfuscated malicious scripts. Future work will focus on expanding the dataset, refining model performance, and incorporating additional deobfuscation techniques to enhance the tool’s accuracy and reliability, thereby advancing the role of applied AI in cybersecurity.
Seoyeong Park
spark43@ncsu.edu
Faculty Sponsor: Munindar P. Singh
Project Title: Meal: Model of Empathy Augmented Logistics for Food Security
Project Abstract
Meal: Model of Empathy Augmented Logistics for Food Security
“Millions globally lack access to nutritious food, experiencing food insecurity. Efforts to address food insecurity seek to provide consumers food that may be rescued (i.e., what warehouses or grocers would otherwise soon discard as unusable), directly donated, or acquired using governmental or other funds. Current approaches produce allocations that optimize global objectives to store and move food efficiently across food banks and to consumers. However, they largely do not take into account consumer preferences and constraints. As a result, the resulting allocations lead to consumers either using foods they don’t care for or discarding such foods, leading to food waste.
We address the problem of equitable food distribution via Meal (Model of Empathy Augmented Logistics for Food Security), a novel approach for allocating food that considers both consumer needs and societal objectives such as reducing food waste and improving equity. Our approach involves introducing a value-aware recommendation system that accommodates two forefront stakeholders’ needs (customers and food banks) and their interactions in allocating food resources. By considering both consumers and food banks and dynamically adapting to changing preferences and resource availability, Meal improves consumer satisfaction and reduces food waste. Specifically, it encourages consumers to be prosocial, i.e., encourages consumers to willingly accept less desirable allocations for others in greater need.
Meal seeks to optimize the allocation strategy toward maximizing the rewards for consumer satisfaction and provider benefit, employing Q-learning. We conduct an experiment on a simulated testbed to verify that Meal finds a way of recommending preferred options and enhancing overall prosociality. Our findings highlight that the right balance for the reward function enhances consumer satisfaction while maximizing provider benefits. We find that Meal is more successful in reducing waste and increasing satisfaction in distributing food items than other models that consider only one side.
In addition, we conducted an empirical study to understand the cognitive factors that affect empathetic behaviors and how prosocial decisions are made throughout interactions. It aims to observe how different human make their decisions to accept the recommendations and foster empathy. In this study, we hypothesize that effective persuasive messages affect the psychological processes of how people react to recommendations beyond simply presenting those. By framing accepting replacements as a collaborative effort toward improving social well-being, we expect to encourage consumers to be less hesitant to accept replacements. Thus, we compared how the participants were satisfied and accepted replacements, depending on whether or not a persuasive message existed.”
Ran Ran
rran@ncsu.edu
Faculty Sponsor: Wujie Wen
Project Title: SpENCNN:Orchestrating Encoding and Sparsity for Fast Homomorphically Encrypted Neural Network Inference
Project Abstract
SpENCNN:Orchestrating Encoding and Sparsity for Fast Homomorphically Encrypted Neural Network Inference
Homomorphic Encryption (HE) is a promising technology to protect clients’ data privacy for Machine Learning as a Service (MLaaS) on public clouds. However, HE operations can be orders of magnitude slower than their counterparts for plaintexts and thus result in prohibitively high inference latency, seriously hindering the practicality of HE. In this paper, we propose a HE-based fast neural network (NN) inference framework–SpENCNN built upon the co-design of HE operation-aware model sparsity and the single-instruction-multiple-data (SIMD)-friendly data packing, to improve NN inference latency. In particular, we first develop an encryption-aware HE-group convolution technique that can partition channels among different groups based on the data size and ciphertext size, and then encode them into the same ciphertext by novel groupinterleaved encoding, so as to dramatically reduce the number of bottlenecked operations in HEconvolution. We further tailor a HE-friendly sub-block weight pruning to reduce the costly HE-based convolution operation. Our experiments show that SpENCNN can achieve overall speedups of 8.37×, 12.11×, 19.26×, and 1.87× for LeNet, VGG-5, HEFNet, and ResNet-20 respectively, with negligible accuracy loss.
Melika Ahmadi Ranjbar
Mahmadi@ncsu.edu
Faculty Sponsor: David Lalush
Project Title: Generative AI Simulation of Anatomically-Realistic Magnetic Resonance Images of the Knee Supporting Automated Quantitative Image Analysis
Project Abstract
Generative AI Simulation of Anatomically-Realistic Magnetic Resonance Images of the Knee Supporting Automated Quantitative Image Analysis
Magnetic Resonance Imaging (MRI) is frequently used in the study of soft-tissue injuries in the knee due to its fine spatial resolution and excellent soft-tissue contrast. Quantitative MRI techniques can also map biochemical properties of the tissue and offer measurements of tissue strain and other quantities. Unfortunately, quantitative analysis often requires highly-accurate segmentation of the important cartilage structures, and this requires manual labeling by a trained human observer. Our project involves the training and evaluation of neural-network models for labeling bones and cartilage in these high-resolution, three-dimensional, multi-channel images. A central problem in developing such models from human-subjects studies is the relatively small dataset compared to most AI-type training environments. While augmentation is often used to pad small datasets, traditional augmentation methods do not provide anatomically-realistic images. This work-in-progress is developing generative AI models that can create distinct, anatomically-realistic knee volumes along with labels of key structures that can be used to train and test AI-based labeling and analysis systems. The model uses a Generative Adversarial Network (GAN) with generator and discriminator components. The generator portion learns to simulate realistic images with feedback from the discriminator, while the discriminator learns to distinguish realistic images from unrealistic ones. The GAN is developed first with two-dimensional slices from MRI image volumes and will later transition to fully 3D images.
Tasmia Shahriar
tshahri@ncsu.edu
Faculty Sponsor: Noboru Matsuda, Shiyan Jiang
Project Title: Enhancing Knowledge-Building in Learning-by-Teaching Environments: Orchestrating Symbolic and Generative AI to drive constructive questions
Project Abstract
Enhancing Knowledge-Building in Learning-by-Teaching Environments: Orchestrating Symbolic and Generative AI to drive constructive questions
We are in the era of computer-supported learning where students interact with a computer system to learn. A paradigm within that direction is Learning-by-Teaching environment. In this environment, students act as tutor to teach computer agents. Such agents are called teachable agent (TA).
Research highlights the benefits of these environments, notably the protégé effect, where students make greater effort to learn for the TAs than they do for themselves. However, students often engage in knowledge-telling behaviors, merely instructing agents without reflecting on their own understanding, which can hinder deeper learning. Conversely, knowledge-building behaviors like providing sense-making explanations and correcting own mistakes yield significant learning benefits.
Building on this foundation, our research utilizes SimStudent, a teachable agent that replicates naturalistic interactions between students and agents by learning from student-provided examples. This process is driven by techniques like inductive logic programming and iterative-deepening depth-first search, allowing SimStudent not only to mimic human learning patterns but also to model student inaccuracies. This capability enables SimStudent to engage in dynamic dialogues, thereby fostering a more engaging and reflective tutoring process.
To further enhance this learning model, we propose to integrate Large Language Models (LLMs) to prompt SimStudent to ask constructive questions that would reduce students’ engagement in knowledge-telling and increase knowledge-building. Our central research question is, can Large Language Models (LLMs) generate adaptive and contextually-driven questions to engage student tutors’ in knowledge-building and thereby, facilitate tutor learning? We propose a novel questioning framework that incorporates three stacked Large Language Models (LLMs). This framework employs a persistent follow-up questioning technique, mirroring the approach teachers use in classrooms. Teachers continuously ask questions to ensure student contributions meet the desired quality, and our model adopts this strategy to promote knowledge-building among student tutors. Our proposed framework requires minimal knowledge-engineering and can be adapted to any procedural problem-solving domains.
To evaluate our proposed framework, we conducted an empirical study involving 35 middle-school students. We divided the students into two groups: one interacted with a SimStudent that utilized our framework to ask follow-up questions, while the other interacted with SimStudent that only posed initial questions to start conversations and did not engage further. In this study, students acted as tutors to teach SimStudent how to solve linear algebraic equations.
Our findings are threefold: (1) Students who engaged with follow-up questions demonstrated greater learning compared to those who did not receive follow-up questions. (2) The follow-up questions significantly enhanced students’ engagement in knowledge-building activities, thereby facilitating conceptual learning across various levels of prior knowledge. (3) Students spent more time responding to questions generated by our framework, which in turn improved their problem-solving accuracy within the Learning-by-Teaching environment.
Utkarsh Sharma
usharma3@ncsu.edu
Faculty Sponsor: Subhashish Bhattacharya
Project Title: Physics Informed Neural Networks Predicting Junction Temperature of Power Devices for PV-Based DC Microgrids
Project Abstract
Physics Informed Neural Networks Predicting Junction Temperature of Power Devices for PV-Based DC Microgrids
This paper proposes a physics-informed neural network model to predict the junction temperature of power devices utilized in the converter that integrates photo voltaic (PV) to the DC Microgrid. The power converter operates at maximum power tracking point mode. The model considers solar irradiance, ambient temperature, output voltage of PV, and switching frequency as inputs. The model is trained using a wide range of real world operating conditions from the National Renewable Energy Laboratory (NREL) database. The importance and utility of the proposed model are presented using two different scenarios. In one of the scenarios, the model is used to predict the duration of different overload conditions that the device can sustain. Such a prediction can help to develop prognostic methods to ensure a device operates within the allowed temperature limits. The other scenario demonstrates the modification in the switching frequency of the converter if the prediction temperature of a device exceeds the allowed limit. This scenario eliminates the need for temperature sensors and again ensures the safe operation of a device.
Divya Sundaresan
dsundar3@ncsu.edu
Faculty Sponsor: Eleni Bardaka; Munindar Singh
Project Title: Empathy in AI: Towards Equitable Microtransit
Project Abstract
Empathy in AI: Towards Equitable Microtransit
This Smart and Connected Communities – Integrative Research Grant (SCC-IRG) Track 1 funded by the National Science Foundation (NSF) seeks to develop and evaluate smart, community-supported solutions for improving efficiency and equity in public microtransit systems. Poor transit service in small cities and towns around the US severely challenges the everyday lives of their many disadvantaged residents. Although public microtransit, which provides point-to-point service in small vehicles, has recently emerged as a promising solution, it remains ineffective at accommodating the rising demand without additional resources (vehicles and drivers). This project seeks to improve system performance equitably, through increased ridesharing and shifting flexible trips to off-peak periods. To this end, it will investigate techniques to motivate microtransit users to act prosocially (volunteer to shift one’s trip time to accommodate the high load of work trips, cooperate with the request to walk more to share a ride with a disabled user, reciprocate after learning that one previously benefited from another user) by evoking feelings of empathy towards other community members. Empathy-building messaging based on real-time user information and powered by artificial intelligence (AI), will enable and motivate prosocial behavior in microtransit at a low cognitive burden while accounting for individual needs and preferences. To increase ridesharing and operational efficiency, microtransit algorithms will be developed for the operation of a first-of-its-kind hybrid system that integrates a commuter program for work and school trips on a fixed, routine schedule with both on-demand and other trips scheduled in advance. These innovations will result in fewer unserved microtransit trip requests and cancelations and therefore lead to quality-of-life improvements, including reduced wage loss and missed medical appointments for riders. The prosocial acts motivated through this research will strengthen community membership, emotional safety, and sense of belonging. This project has the potential to benefit the thousands of small US communities that lack access to employment, health care, and other critical destinations. This research will create new paradigms for facilitating prosocial behavior in sociotechnical systems, moving away from traditional pricing mechanisms and incentives. This project engages with both governmental and nongovernmental organizations to understand stakeholder needs and improve stakeholder acceptance of the technology and will advance our understanding of the contributions of community-based organizations and education in the success of smart and connected communities.
Benyamin T. Tabarsi
btaghiz@ncsu.edu
Faculty Sponsor: Tiffany Barnes, Dongkuan (DK) Xu
Project Title: MerryQuery: A Trustworthy LLM-Powered Tool Providing Personalized Learning Support for Educators and Students
Project Abstract
MerryQuery: A Trustworthy LLM-Powered Tool Providing Personalized Learning Support for Educators and Students
Large Language Models (LLMs) have sparked both enthusiasm and concern in educational settings. While they offer new possibilities for learning, challenges such as student over-reliance, lack of educator control, and the risk of hallucinations are fueling a divide among educators. To address these issues, we introduce MerryQuery, an AI-powered educational assistant designed to empower teachers and enhance student learning outcomes. Leveraging retrieval-augmented generation (RAG), MerryQuery generates personalized responses based on course-specific materials—such as syllabi, slides, and forum discussions—while prioritizing transparency by citing sources. The system is adaptable across subject areas, allows educators to control the completeness of responses, and features tools for monitoring student interactions. The prototype has already finished and will be trialed in Fall 2024 in Dr. Lina Battestilli’s course, CSC 113 – Introduction to Computing – MATLAB, to gather feedback from real-world use.
MerryQuery’s development focuses on three core objectives: creating LLM-powered agents for personalized content generation, implementing real-time feedback mechanisms, and automating routine administrative tasks while ensuring content trustworthiness. Through rigorous pilot studies, we aim to refine the system and assess its impact on student engagement and learning outcomes. Open-sourced and supported by community engagement through workshops and webinars, MerryQuery is designed to bridge the gap between advanced AI technology and education, providing a scalable, reliable solution that meets the needs of diverse educational environments. This project is guided by Dr. Tiffany Barnes and Dr. DK Xu, experts in AI-driven educational technologies.
Lixing Zheng
lzheng26@ncsu.edu
Faculty Sponsor: Wujie Wen
Project Title: DeepN-JPEG: a deep neural network favorable JPEG-based image compression framework
Project Abstract
DeepN-JPEG: a deep neural network favorable JPEG-based image compression framework
As one of most fascinating machine learning techniques, deep neural network (DNN) has demonstrated excellent performance in various intelligent tasks such as image classification. DNN achieves such performance, to a large extent, by performing expensive training over huge volumes of training data. To reduce the data storage and transfer overhead in smart resource-limited Internet-of-Thing (IoT) systems, effective data compression is a “must-have” feature before transferring real-time produced dataset for training or classification. While there have been many well-known image compression approaches (such as JPEG), we for the first time find that a human-visual based image compression approach such as JPEG compression is not an optimized solution for DNN systems, especially with high compression ratios. To this end, we develop an image compression framework tailored for DNN applications, named “DeepN-JPEG”, to embrace the nature of deep cascaded information process mechanism of DNN architecture. Extensive experiments, based on “ImageNet” dataset with various state-of-the-art DNNs, show that “DeepN-JPEG” can achieve ~3.5x higher compression rate over the popular JPEG solution while maintaining the same accuracy level for image recognition, demonstrating its great potential of storage and power efficiency in DNN-based smart IoT system design.
Electrical and Computer Engineering
Devadharshini Ayyappan
dayyapp@ncsu.edu
Faculty Sponsor: Edgar Lobaton
Project Title: Enhancing Water Purification through AI: Monitoring Mussel Behavior for Scalable Environmental Solutions
Project Abstract
Enhancing Water Purification through AI: Monitoring Mussel Behavior for Scalable Environmental Solutions
Water quality remains a pressing issue in many regions, with pollutants such as heavy metals posing significant risks. Detecting these toxins, like mercury, in water bodies is challenging due to their often invisible nature. However, mussels, known for their role as bioindicators and filter feeders, offer a natural solution. Their ability to purify water by filtering out contaminants makes them an ideal subject for study. This project leverages the power of Artificial Intelligence (AI) and Machine Learning (ML) to monitor mussel behavior, specifically their gaping behavior, to better understand and enhance the process of water decontamination.
The primary focus of this research is on the application of AI and ML to analyze mussel behavior in a controlled environment. By continuously monitoring the gaping behavior of mussels, we can gain valuable insights into their role in water purification. The study aims to determine the extent to which mussels can be used for large-scale toxin removal, potentially benefiting entire ecosystems, such as rivers, by naturally filtering out harmful substances.
The integration of AI and ML is at the heart of this project. We utilize advanced algorithms to process and analyze data collected from sensors that monitor various environmental factors, such as water temperature, pH levels, and the heartbeat of the mussels. This sensor data, combined with the mussels’ gaping behavior, provides a rich dataset that can be used to build predictive models. These models can help us understand the conditions under which mussels are most effective at purifying water and how their behavior correlates with the presence of specific pollutants. To further enhance our analysis, we employ cutting-edge 3D reconstruction techniques. Initially, 2D images of different types of mussels are collected and annotated. These images are then used to create detailed 3D models through techniques such as Gaussian Splatting and Neural Radiance Fields (NeRF). These 3D models allow us to estimate the orientation and position of mussels in new underwater settings, providing a more accurate representation of their natural behavior. This spatial information is crucial when combined with the AI/ML models that analyze their gaping behavior, as it enables us to develop a more holistic understanding of their filtering process.
In the broader context of applied AI in engineering and computer science, this research underscores the transformative potential of AI and ML when applied to environmental challenges. By integrating sensor data, advanced imaging techniques, and predictive modeling, we can create intelligent systems that contribute to a cleaner, healthier environment, showcasing the vital role of AI and ML in driving innovation across diverse fields.
Sina Baghbanijam
sbaghba@ncsu.edu
Faculty Sponsor: Tianfu Wu
Project Title: Strawberry Daughter Plant Property Prediction Utilizing Artificial Intelligence
Project Abstract
Strawberry Daughter Plant Property Prediction Utilizing Artificial Intelligence
Strawberry plant growth monitoring and yield prediction have traditionally relied on manual observation, expensive specialized equipment, and outdated algorithms, posing challenges to efficiency, scalability, and accuracy. To address these limitations, we have developed a comprehensive dataset of 2224 strawberry daughter plants, using a low-cost stereo vision setup composed of two Raspberry Pi cameras. This setup captures three images per camera for each plant, providing six images per sample. Each plant in the dataset is labeled with key agronomic attributes, including total leaf area, largest petiole length (cm), fresh mass, dry mass, and average crown diameter, enabling a multifaceted analysis of plant growth.
To predict these crucial plant attributes, we have developed multiple deep learning models, leveraging convolutional neural networks (CNNs) and Vision Transformer (ViT) to extract and process visual information from the dataset. A unique contribution of this work is the implementation of a late fusion approach that effectively combines the features from all six images for each sample, resulting in more accurate predictions. The late fusion mechanism aggregates features from each camera’s view, allowing the model to account for different perspectives and enhance overall prediction accuracy.
Zach Bloom
Zabloom@ncsu.edu
Faculty Sponsor: Michael Kudenov
Project Title: High-Throughput Classification and Quantification of Skinning Phenotype in Sweet Potatoes
Project Abstract
High-Throughput Classification and Quantification of Skinning Phenotype in Sweet Potatoes
Sweetpotatoes (Ipomoea batatas) (SPs) have become an important crop in many parts of the world. The tuberous root is valued for its vibrant colors and sweet flavor profile, making it a versatile ingredient in many culinary dishes. However, mechanical harvesting of SPs is not possible due to their fragile skins (periderm), especially when compared to white potatoes. Thus, harvesting is highly labor-intensive and involves hand harvesting in the field. Subsequent rough handling, improper or incomplete curing, or impacts on the production line cause skinning (A_s) that results in a quality control issue during packing. In this paper, we trained a convolutional neural network to perform semantic segmentation to help farmers make informed decisions about when to switch fields during packing, based on product A_s. The developed system featured a machine vision camera mounted above a motorized conveyor belt at the start of the production line. A computer vision pipeline, using a ResNet50 backbone and DeepLabV3+ semantic segmentation model, was developed to analyze SPs for the A_s phenotype. The model was used to calculate the percentage of A_s on each SP and verify daily production trends that were identified by our collaborating stakeholder. Validation was conducted on six days’ worth of production data in which a A_s related field-switch decision was made by the stakeholder. In total, 2,017,199 SP instances were analyzed. The results indicated an RMSE of 0.55%, an R² value of 0.84, a testing accuracy of 80.00% for predicting the A_s class, and an overall instance grading accuracy of 92.70%. Overall, the trained model demonstrated the ability to detect percent skinning (PS) in SPs at an industrial food processing scale, suggesting a potential improvement to the grading process that could benefit farmers at all levels.
James Boulton
jaboulto@ncsu.edu
Faculty Sponsor: Ki Wook Kim
Project Title: Predicting 3D Magnetic Topological Insulators and Semimetals with Machine Learning
Project Abstract
Predicting 3D Magnetic Topological Insulators and Semimetals with Machine Learning
This project uses machine learning models to determine which magnetic molecules are topological insulators, semimetals, trivial insulators, and trivial metals. The search for magnetic topological insulators and semimetals is important for the application of spintronic devices. In the past, identifying topological insulators and semimetals has required time-intensive Density Functional Theory (DFT) calculations followed by a Hamiltonian model using Maximally Localized Wannier Functions (MLWF). A machine learning (ML) model should be able to identify topological insulators and semimetals much quicker. The materials of interest in this project are AxByCz compounds where A = Cobalt, Chromium, Europium, Nickel, Vanadium, B = Bismuth, Antimony, and C = Tellerium or Selenium.
The data used for this work originates from Materials Project, Aflow, the Topological Materials Database, and the Topological Magnetic Materials Database. Base material properties including whether materials were metals or topological were obtained from these databases. The atomic properties were added in python to the base material data retrieved from the above databases. The average, difference, maximum, minimum, sum, and variance were evaluated for all materials.
The algorithm for this work consists of utilizing gradient boosted trees after initial possible data reduction by Sure-Independence-Screening Sparsifying Operator (SISSO) and principal component analysis (PCA). Since the identification of topological insulators and semimetals is known to be a highly non-linear problem, linear regression or linear based algorithms are not appropriate. This project evaluates the use of both a Random Forest of trees and Gradient Boosted trees. If these prove to be insufficient, neural networks will be considered.
Currently, I am using three XGBoost models coded in python. The first XGBoost model predicts whether the material is an insulator or a metal. For materials predicted as insulators, the second model predicts whether that material is a topological insulator or a trivial insulator. For materials predicted as metals, the third model predicts whether that material is a semimetal or a trivial metal.
Before creating training sets, the data was balanced to have equal numbers of the major class. For topological insulators, it was also balanced to have equal classes per number of atoms in a molecule. The data was then split into a training set, a validation set and a test set in a 80:10:10 ratio for each ML model. After training, a high level of accuracy was achieved for the topological model (95.7%). The metal/nonmetal model achieved 88.0% accuracy. The semimetal ML model was much less accurate (79.9%). These final models were used to predict which of our materials of interest were either topological insulators or semimetals.
Verification of these results with DFT and MLWFs has begun. Five of the materials of interest have been confirmed as topological insulators so far. However, only one of the predicted semimetals of the materials of interest has been shown to be a semimetal. Therefore, further work is being done on the semimetal model. A neural network semimetal model is in progress.
Gunavaran Brihadiswaran
gbrihad@ncsu.edu
Faculty Sponsor: James Tuck
Project Title: Deep Learning-Driven DNA-DNA Binding Predictions for Efficient DNA-Based Data Storage
Project Abstract
Deep Learning-Driven DNA-DNA Binding Predictions for Efficient DNA-Based Data Storage
The exponential increase in global data and the limitations of existing archival storage technologies in meeting the demand–such as density scalability, limited longevity, and sustainability–have driven the search for an alternative storage medium. Synthetic DNA is widely regarded as a promising solution due to its high density, durability, sustainability, and low maintenance costs. A crucial aspect of DNA data storage is the accurate prediction of whether two DNA sequences will bind, as it facilitates the generation of more primers for file indexing, minimizes off-target bindings, and opens avenues for new functionalities. Furthermore, accurate prediction of DNA binding is equally important in other fields such as genomics, molecular biology, and synthetic biology. Traditionally, decisions regarding the binding potential of two DNA sequences have relied on complex thermodynamic computations. Although popular tools based on thermodynamics exist to analyze DNA behavior, they struggle to scale effectively in large systems with millions of sequences due to the exponential increase in computational requirements. Several machine-learning approaches utilizing in silico pairwise sequence data have been proposed to address this scalability challenge. However, our experiments reveal that models trained on in silico-generated data perform poorly in predicting DNA interactions in actual wet lab experiments. We present a convolutional neural network (CNN) model trained on data from wet lab experiments designed to determine which sequences will bind. A library of randomized 20-mer overhang sequences was annealed to 5 different biotinylated bead primer sequences. The biotinylated beads primers, bound or not, were subsequently pulled out using a streptavidin magnetic bead. After pullout, library strands bound to the bead primer were eluted to form the bound library, and the library strands that were left behind and did not bind to the bead primer molecules formed the unbound library. The bound and unbound libraries were sequenced to obtain a set of sequences for training our model. Each instance consists of a bead primer, a sequence from the bound or unbound library, and a label indicating if it was bound or unbound. The combined dataset comprises approximately 2.5 million sequence pairs, evenly split between the bound and unbound target classes. These sequences were one-hot encoded to generate the input matrices for our model. We used 80% of the data for training, 10% for validation, and 10% for testing. The CNN model was implemented using the PyTorch Lightning framework and trained on a GPU. The hyperparameters were optimized using the ASHA algorithm with the help of the Ray Tune library. Our model achieved an accuracy of 88% in predicting the bound and unbound sequence pairs, outperforming the state-of-the-art thermodynamics-based tool by over 20%.
Khoa Do
kddo@ncsu.edu
Faculty Sponsor: Fred Livingston
Project Title: AI-Driven Cyber-Physical Systems: Enhancing Post-Processing in Advanced Manufacturing
Project Abstract
AI-Driven Cyber-Physical Systems: Enhancing Post-Processing in Advanced Manufacturing
This research presents a state-of-the-art cyber-physical system to transform post-processing in advanced manufacturing. The system integrates robotics, real-time 3D perception, and sophisticated motion planning algorithms with applied AI, particularly in vision processing, to achieve exceptional precision and efficiency. By utilizing AI deep learning models like YOLOv8 for pose detection, in combination with traditional computer vision algorithms such as Perspective-n-Point (PnP) and the Robot Operating System (ROS), the system enables real-time 3D pose detection of manufacturing parts, crucial for accurate robotic manipulation during post-processing.
The research employs a dual-environment approach: a real robotic environment and a digital twin environment powered by NVIDIA Isaac Sim. In the real robotic setup, UR5 robot manipulators executes post-processing tasks such as support structure removal or surface finishing (like painting, welding, polishing, and so on), guided by real-time data-driven AI vision systems and IoT technologies. This ensures high-precision parts handling in a dynamic manufacturing environment, significantly reducing errors and improving overall efficiency.
Simultaneously, a digital twin environment is created using NVIDIA Isaac Sim to mirror real-world robotic operations. This high-fidelity simulation allows for rapid development, testing, and optimization of the system in a virtual space. The digital twin accelerates the prototyping process, reduces development costs, and provides a safe environment to test new algorithms and refine the system’s performance. It also serves as a training ground for AI models, enabling the simulation of diverse scenarios that may be challenging to replicate in the real world.
This integrated approach ensures that advancements made in the virtual environment can be seamlessly transferred to the physical setup, enhancing the system’s robustness and adaptability. The research sets a new benchmark for efficiency and precision in post-processing for advanced manufacturing, offering a smarter, more adaptive solution for the industry.
Pranshav Gajjar
pranshav.gajjar@gmail.com
Faculty Sponsor: Vijay K Shah
Project Title: Leveraging Large Language Models for Open Radio Access Networks: Introducing ORAN-Bench-13K and ORANSight
Project Abstract
Leveraging Large Language Models for Open Radio Access Networks: Introducing ORAN-Bench-13K and ORANSight
Large Language Models (LLMs) can revolutionize how we deploy and operate Open Radio Access Networks (O-RAN) by enhancing network analytics, anomaly detection, and code generation and significantly increasing the efficiency and reliability of many O-RAN tasks. We present ORAN-Bench-13K, the first comprehensive benchmark designed to evaluate the performance of Large Language Models (LLMs) within the context of O-RAN. Our benchmark comprises 13,952 meticulously curated multiple-choice questions from 116 O-RAN specification documents. We leverage a novel three-stage LLM framework, and the questions are categorized into three distinct difficulties to cover a wide spectrum of ORAN-related knowledge. We thoroughly evaluate the performance of several state-of-the-art LLMs, including Gemini, Chat-GPT, and Mistral. Additionally, we propose ORANSight, a Retrieval-Augmented Generation (RAG)-based pipeline that demonstrates superior performance on ORAN-Bench-13K compared to other tested closed-source models. ORANSight can be incorporated as a Generative AI system to enhance various tasks like automated RAN testing, Code Generation, real-time analysis, and RAN control. This work aligns with the AI RAN alliance’s objectives to integrate advanced AI technologies into RAN systems. It supports the O-RAN alliance’s objective to create open, intelligent, and interoperable RAN systems. We are also working towards creating ‘TestORAN’ a holistic benchmark for evaluating O-RAN testing tools. It replicates possible issues and errors encountered in a real system and leverages ORANSight to compute semantically accurate bugs.
Abiodun Ganiyu
aganiyu@ncsu.edu
Faculty Sponsor: Vijay Shah
Project Title: AI5GTest: AI-Enabled Testing Method for Open Radio Access Networks Components
Project Abstract
AI5GTest: AI-Enabled Testing Method for Open Radio Access Networks Components
The advent of Open Radio Access Networks (O-RAN) has brought numerous benefits to the telecommunication industry promoting interoperability and fostering innovation through its open interfaces and modular components. Its disaggregated nature facilitates multi-vendor collaboration, enabling both large and small vendors to design and deploy components in the cellular network industry. Given the diversity of the software and hardware vendors involved, it is important to rigorously test these components before they are deployed to the market. Our project introduces an AI-enabled testing framework that revolutionize the validation of O-RAN components, addressing the limitations of traditional manual testing methods. Conventional methods, which involve manually running tests and validating signaling messages, are not only time-consuming but also susceptible to human error. To address these challenges, we propose a novel methodology leveraging AI-driven testing framework. Central to this framework is a Retrieval-Augmented Generation (RAG) pipeline integrated with a Large Language Model (LLM). This tool automates the process of cross-referencing O-RAN and 3GPP standards, generating expected procedural flows, and validating real-time signaling data against these standards. By employing AI, the framework will ensure efficient and accurate testing, reducing likelihood of human errors and significantly enhancing the reliability of O-RAN components validation. As the O-RAN system continues to expand, with over 14,560 3GPP documents and 116 O-RAN documents to consider, our proposed framework aims to offer a scalable solution to ensure O-RAN components from various vendors meet the highest standards of performance, interoperability and security.
Emily Garceau
egarcea@ncsu.edu
Faculty Sponsor: Alper Bozkurt, Anders Huseth, James Reynolds
Project Title: AI Supported Hartstack Pheromone Traps for Digitized Moth Counting
Project Abstract
AI Supported Hartstack Pheromone Traps for Digitized Moth Counting
In the face of increasing agricultural challenges, the use of AI in pest management is becoming essential for sustainable farming. This project focuses on enhancing Hartstack pheromone traps with advanced AI-driven digitized counting technology to automate the monitoring of Helicoverpa zea (Boddie) moth populations, one of the most significant crop pests in the eastern United States.
The cross-college collaboration between the Integrated Bionic MicroSystems Laboratory (COE, Electrical Engineering) and the Precision Pest Ecology Laboratory (CALS, Entomology) aims to address the limitations of traditional moth traps by integrating infrared sensors and environmental data loggers. These sensors capture the presence of moths as they enter the traps, while environmental sensors record local conditions such as temperature, humidity, wind speed, and atmospheric pressure.
During the summers of 2022 and 2023, these traps were deployed across fields in North Carolina, creating a large spatiotemporal dataset. Analyzing moth detections from the infrared (IR) sensors presents difficulties due to false positives caused by erratic moth flight paths and other small insect species entering the traps. Additionally, recording moth detections as timestamps creates a rudimentary binary signal (detected/not detected), which limits available analysis techniques. To overcome these challenges, several machine learning (ML) approaches have been applied, such as kernel density estimation to convert the binary signal into a probability curve, windowing detection data to examine common patterns, and sequential filtering techniques.
The best performance so far has been achieved using custom-designed filters and preprocessing techniques, which find a strong linear relationship between ground truth detections and trap detections. The most successful filtering method combines a delta filter and a parallel filter applied sequentially to optimize moth detection accuracy. The delta filter removes closely timed sensor triggers to address multiple detections from single moths, improving the correlation between true and predicted moth counts from R² = 0.41 (raw data) to R² = 0.60. The parallel filter then checks if the remaining triggers align between the pair of sensors, further enhancing the correlation to R² = 0.70. The combined parallel-delta filter, refined through grid search and MATLAB’s optimization, achieves the best performance when predicting the true moth count, with an R² = 0.75.
The outcomes of this project have significant implications for agriculture in the United States. By providing accurate data on moth populations, the system allows farmers to optimize pest control strategies, reducing the need for blanket pesticide applications and thereby promoting more sustainable farming practices.
Vahid Jebraeeli
vjebrae@ncsu.edu
Faculty Sponsor: Hamid Krim
Project Title: Applied AI solutions to data processing, environmental prediction, and industrial safety
Project Abstract
Applied AI solutions to data processing, environmental prediction, and industrial safety
Our research activities in VISSTA Laboratory focus on exploring novel and adapted AI solutions to real-world problems and mitigating limitations of existing methodologies. Our approach to solving problems unveils fundamental concepts with broad algorithmic impact on vast application domains. We will illustrate recent research in our Lab, namely improving predictions for Tropical cyclone activity by leveraging multi-modal information fusion, and on anomaly detection in nuclear power plants by transfer learning, and on dataset condensation & expansion techniques for limited training data availability scenarios.
Tropical Cyclone Activity Prediction: TC activity prediction, as a statistical outlier, is notoriously complex. The immeasurably complex interactions of the atmosphere and the ocean occur at multiple spatio-temporal scales and make the selection of appropriate predictors difficult and strongly reliant on good domain knowledge. Such a non-trivial selection approach is not guaranteed to consistently capture all the latent non-linear dynamics, making the realization of a comprehensive model highly challenging. To go beyond the limited accounting for the vast environmental factors and the resulting poor and even absent interpretability of current ML models in this area, we bridge the gap between the SOTA approaches of weather-science and modern ML by proposing an efficient and physics-based multi-modal information fusion to predict long- as well as short-term TC activity. This affords longer forecast periods with good accuracy in comparison to current models.
Anomaly Detection in Nuclear Power Plants: The scarcity of operational data in nuclear power plant operations, especially during transient conditions like power fluctuations, makes anomaly detection highly challenging. Traditional methods rely on abundant stable full-power data and the ensuing information short-fall yields increased false alarms or missed detections, compromising system safety and reliability. We address this problem by a transfer learning-based anomaly detection method by extracting informative features from stable full-power data using LSTM autoencoders, followed by dimensionality subspace-based adaptation. Subspace matching is subsequently employed to harmonize the source domain (stable conditions) with the target domain (transient conditions), via an optimal selection of the best-matching feature subset. This approach enables effective knowledge transfer, significantly improving the accuracy and reliability of anomaly detection, particularly in the scarce data scenario during transient conditions.
Dataset Condensation and Expansion: Underlying many AI solutions is the assumption of abundant large datasets for training. However, in real-world environments, we face limited data availability. To address these scenarios, we explore Dataset Condensation and Expansion duality. By invoking Koopman space data representation, we develop Koopcon, an Autoencoder-based model exploiting this representation to compress large datasets into compact non-linear forms, benefiting from implicit and neuro-inspired predictive coding. This formalism leads to an Expansive Synthesis model tackling data scarcity by generating large-scale datasets from minimal samples. This approach uses expander graph mappings and feature interpolation, exploiting neural networks’ latent space and Koopman operator theory to realize dataset dimension expansion. Experiments show classifiers trained on condensed or expanded datasets achieve performance comparable to original datasets. By enabling effective data handling in resource-constrained environments and augmenting training data, these models pave the way for smarter learning paradigms across ML applications.
Yi Hu
yhu28@ncsu.edu
Faculty Sponsor: Ning Lu
Project Title: Generative AI for Power System Data Analysis
Project Abstract
Generative AI for Power System Data Analysis
The increasing complexity of power systems, driven by renewable energy integration and smart grid technologies, is placing unprecedented demands on data availability and quality. However, the scarcity of high-quality, comprehensive datasets poses significant challenges to advancing artificial intelligence (AI)-driven solutions in power systems. My research tackles this problem by leveraging state-of-the-art AI models—specifically Generative Adversarial Networks (GANs), BERT, Transformers, and fine-tuned large language models (LLMs)—to address critical issues related to synthetic data generation, missing data restoration, demand response baseline estimation, and anomaly detection.
Data scarcity remains one of the biggest hurdles in power system analysis. Real-world datasets are often incomplete, confidential, or difficult to obtain, which restricts the development and deployment of effective AI models. To combat this, my work applies GANs and Transformer-based models to generate synthetic load profiles that accurately capture spatial-temporal correlations across households and time periods. These synthetic datasets replicate the characteristics of real data, overcoming both privacy concerns and the challenges posed by limited data availability. By providing a reliable source of high-quality synthetic data, this work directly supports downstream tasks such as missing data restoration, baseline estimation, and anomaly detection.
In addition to generating synthetic data, my research also focuses on restoring missing data, which is crucial for maintaining the integrity of power system datasets. My research employs BERT-based models and fine-tuned LLMs to restore missing load data, predicting missing values by learning contextual dependencies within the data. The BERT-based model ensures that restored data maintains the structural properties of the original dataset, achieving the highest accuracy observed so far. Meanwhile, the fine-tuned LLM achieves comparable accuracy but requires significantly less training data and computing resources, another solution to data scarcity by reducing data need. These techniques are particularly valuable for demand response baseline estimation, where accurate reconstructions of consumption patterns are essential for evaluating demand-side management strategies. By restoring incomplete data and reducing data needs, this research enhances the quality and utility of available datasets, thereby mitigating the impact of data scarcity.
Anomaly detection is a crucial area where AI can significantly improve power system reliability. My research utilizes semi-supervised GAN-based models to identify anomalies in load data by detecting deviations from typical patterns that could indicate equipment failures. This method reduces the reliance on labeled datasets, making it a more efficient approach for identifying potential issues. Through early failure detection and accurate classification, this approach strengthens the resilience and security of power systems.
Overall, my research advances the application of Generative AI in power systems by addressing the critical issue of data scarcity through innovative solutions in data generation, restoration, baseline estimation, and anomaly detection. By integrating GANs, BERT, Transformers, and fine-tuned LLMs, this work contributes to the development of more reliable, efficient, and resilient power systems, with significant implications for both academia and industry.
Riddhi Khatua
rkhatua@ncsu.edu
Faculty Sponsor: Aranya Chakrabortty
Project Title: Power Flow Optimization and Stability Analysis using Physics Informed Neural Networks
Project Abstract
Power Flow Optimization and Stability Analysis using Physics Informed Neural Networks
With the increasing penetration of inverter based resources in the modern day power grid, several stability issues arise due to the uncertainties associated with their integration. These facilitate the development of robust data-driven algorithms that can adapt to dynamic changes without requiring the system to go offline. A physics-informed neural network has been trained to predict H2-norm optimized power flow setpoints by performing parametric optimization of the linearized grid transfer function. The proposed algorithm offers flexibility to various incoming disturbances without requiring offline retraining. Network physics have been embedded in the NN loss function through a physics regularization term. The risk curves of the renewables have been considered as an additional optimization term in the objective function while performing gradient descent in the model based code. Training scenarios have been generated by uniform sampling around the nominal dispatch, which acts as the input to the model based code. The proposed methodology has been tested on a 16 machine, 82 bus modified New York Academic Model with 7 wind farms integrated into the power system. Neural network predictions have been passed through additional checks to ensure that they make sense from a power system point of view as well as being numerically close to the model based values. It has been observed that the data-driven algorithm offers enhanced stability indices (small-signal and transient) as compared to traditional cost optimal setpoints. Moreover, stability indices calculated from data-driven and model-based setpoints are numerically accurate despite the data-driven solver being a one-shot prediction mechanism (as compared to model based H2-norm optimized power flow which follows iterative gradient descent). The novelty of this work lies in the fact that a complex multi-objective optimization problem involving power system dynamics has been solved with the usage of physics informed neural networks.
Donghyeon Koh
dkoh2@ncsu.edu
Faculty Sponsor: W. Rhett Davis
Project Title: PPA Prediction using Graph Neural Network
Project Abstract
PPA Prediction using Graph Neural Network
Power, performance, and area are the main variables that decide integrated circuit (IC) performance. PPA cannot be determined during the design process as it takes a long time to compute. Therefore, predicting PPA in the early stage of the design will help designers optimize the design in the process.
The transfer learning approach [1] with neural network (NN) used a small number of samples to retrain the model to optimize the model to predict different designs. The model prediction achieved sufficient accuracy in predicting areas. However, the accuracy of critical path delay prediction was comparably low even after the transfer learning to optimize the model to the design. Also, this approach showed limited design variation for prediction which implies different PPA model is needed to expand the design variation for prediction.
We then attempted a new PPA model named meta-transfer learning which trains from individual input samples more closely than regular transfer learning. The meta-transfer learning is separated into two parts: inner optimization and outer optimization. During the inner optimization, a single sample from the datasets is used multiple times using the feedback loop, strongly optimizing the PPA model to that specific sample. Meta-transfer learning showed increased accuracy across PPA metric but still exhibit limitation in predicting various designs. Specifically, if the statistical distance between the initial design and the retraining design is greater, the model shows lower accuracy, indicating that meta-transfer learning is still dependent on the initial design used to train the model.
This is when the graph neural network (GNN) model using graph dataset came to our interest. The GNN model introduced in [2] predicts the maximal arrival time by using a directed acyclic graph (DAG) as their dataset. However, the maximal arrival time prediction is executed with small designs and the dataset is collected before the synthesis. This prevents the design from optimizing in the process of the synthesis but will show limitations on bigger designs. The research from [3] also used DAG as their dataset and proposed a directed acyclic graph neural network (DAGNN) model to predict the longest path of abstract syntax trees (ASTs). The research demonstrated a high accuracy of 99.93% compared to the graph convolutional network (GCN) model’s prediction accuracy of 95.55%.
Thus, we propose using a new method of GNN model with transfer learning to accurately predict the PPA metrics for a wide variety of designs.
[1] Francisco, Luis, Paul Franzon, and W. Rhett Davis. “”Fast and Accurate PPA Modeling with Transfer Learning.”” 2021 ACM/IEEE 3rd Workshop on Machine Learning for CAD (MLCAD). IEEE, 2021.
[2] Lopera, Daniela Sánchez, Ishwor Subedi, and Wolfgang Ecker. “”Using Graph Neural Networks for Timing Estimations of RTL Intermediate Representations.”” 2023 ACM/IEEE 5th Workshop on Machine Learning for CAD (MLCAD). IEEE, 2023.
[3] Thost, Veronika, and Jie Chen. “”Directed acyclic graph neural networks.”” arXiv preprint arXiv:2101.07965 (2021).
Xingjian Li
xli228@ncsu.edu
Faculty Sponsor: Lirong Xiang
Project Title: Photorealistic Arm Robot Simulation for 3D Plant Reconstruction and Automatic Annotation using Unreal Engine 5
Project Abstract
Photorealistic Arm Robot Simulation for 3D Plant Reconstruction and Automatic Annotation using Unreal Engine 5
Robotics paired with computer vision are widely used in precision agriculture. Simulations are critical for safety and performance estimation by verifying their routine in a virtual world before real-world testing and deployment. However, many simulators used in agricultural robots lack photorealism in their virtual worlds compared to the real world. We implemented Unreal Engine 5 (UE5) and the Robot Operating System (ROS) to develop a robot simulator tailored to agricultural tasks and synthetic data generation with RGB, segmentation, and depth images. We designed a method for assigning multiple segmentation labels within a single plant mesh. We experimented with a semi-spherical routine for two robot arms to perform 3D point cloud reconstruction across 10 plant assets in indoor and outdoor environments. We showed our simulator produces accurate segmentation images and reconstruction. We extend our results with Neural Radiance Field (NeRF) and 3D Gaussian Splatting (3DGS) reconstructions.
Roghieh Mahdavihaji
rmahdav2@ncsu.edu
Faculty Sponsor: Duel-Hallen
Project Title: ML-Aided mmWave/sub-THz Blockage Prediction in Realistic Outdoor Mobile Systems
Project Abstract
ML-Aided mmWave/sub-THz Blockage Prediction in Realistic Outdoor Mobile Systems
The surge in mobile data consumption, driven by the proliferation of smartphones and high-bandwidth applications, poses significant challenges for wireless service providers as they grapple with a global bandwidth shortage. Utilization of millimeter wave (mmWave) and sub-THz frequencies can potentially boost the throughput of wireless communication systems since they provide much wider available bandwidth than the sub-6 GHz frequencies. However, there are inherent challenges to effective deployment of mmWave/sub-THz technology. In particular, line-of-sight (LoS) blockage by static or moving physical objects can severely limit performance of these systems due to more abrupt variation of the received signal strength (RSS) caused by weaker diffraction and greater penetration loss than for sub-6GHz frequencies. To address this problem, we previously proposed to employ MiniRocket machine learning (ML) method to forecast approaching blockage sufficiently far ahead to find a new beam or perform a handover between base stations. MiniRocket ML algorithm provides early warning (EW) of LoS mmWave/sub-THz blockage by exploiting diffraction-induced pre-blockage oscillation patterns of the received signal strength (RSS). This algorithm is less complex and much faster than deep learning (DL) and recurrent neural network (RNN) models and thus is more suitable for real-time applications. The MiniRocket methods employs (10,000) convolutional kernels of length 12 with random weights and dilation to identify the diffraction-induced features that warn about the approaching blockages and to distinguish them from other signal features. Due to the utilization of numerous convolutional kernels with different dilation and padding, MiniRocket classifier is suitable for extraction of the oscillation patterns at various frequencies. To create the mmWave signal datasets for training and testing the ML, we utilize our low-complexity physics-based simulation tool incorporating Fresnel diffraction and image sources. Unlike most works in the literature, our work does not assume specific topology, directions, or speeds and does not use simplistic simulation models. Moreover, our approach relies solely on in-band mmWave or sub-THz signal measurements and does not require sub-6 GHz observations or cameras. Since our previous work did not include multiple antennas at the transmitter and receiver and did not model reflectors in the environment, we modify the physical model to include these realistic features. We analyze the performance of the ML-aided early-warning of blockage (ML-EW) algorithm for diverse reflectors and antenna arrays placed at the base station (BS) and user equipment (UE) over a range of mmWave and sub-THz frequencies. Our results indicate that ML-EW performance accuracy approximates or exceeds 90% for highway UE, blocker, and reflector speeds, multiple-input-multiple-output (MIMO) systems, and frequencies in mmWave/sub-THz range. It enables warning about the upcoming blockage 100 ms ahead, or around ten 5G frames, for highway speeds, providing sufficient time for a proactive response.
Sreejeet Maity
smaity2@ncsu.edu
Faculty Sponsor: Aritra Mitra
Project Title: Robust Algorithms for Adversarial Reinforcement Learning
Project Abstract
Robust Algorithms for Adversarial Reinforcement Learning
Recently, there has been a surge of interest in analyzing the non-asymptotic behavior of model-free reinforcement learning algorithms. However, the performance of such algorithms in non-ideal environments, such as in the presence of corrupted rewards, is poorly understood. Motivated by this gap, we investigate the robustness of the celebrated Q-learning algorithm to a strong-contamination attack model, where an adversary can arbitrarily perturb a small fraction of the observed rewards. We start by proving that such an attack can cause the vanilla Q-learning algorithm to incur arbitrarily large errors. We then develop a novel robust synchronous Q-learning algorithm that uses historical reward data to construct robust empirical Bellman operators at each time step. Finally, we prove a finite-time convergence rate for our algorithm that matches known state-of-the-art bounds (in the absence of attacks) up to a small inevitable $O(\varepsilon)$ error term that scales with the adversarial corruption fraction $\varepsilon$. Notably, our results continue to hold even when the true reward distributions have infinite support, provided they admit bounded second moments.
Saad Masrur
smasrur@ncsu.edu
Faculty Sponsor: Ismail Guvenc
Project Title: Energy-Efficient Sleep Mode Optimization of 5G mmWave Networks Using Deep Contextual MAB
Project Abstract
Energy-Efficient Sleep Mode Optimization of 5G mmWave Networks Using Deep Contextual MAB
Millimeter-wave (mmWave) networks, integral to 5G communication, offer a vast spectrum that addresses the issue of spectrum scarcity and enhances peak rate and capacity. However, their dense deployment, necessary to counteract propagation losses, leads to high power consumption. An effective strategy to reduce this energy consumption in mobile networks is the sleep mode optimization (SMO) of base stations (BSs). In this paper, we propose a novel SMO approach for mmWave BSs in a 3D urban environment. This approach, which incorporates a neural network (NN) based contextual multi-armed bandit (C-MAB) with an epsilon decay algorithm, accommodates the dynamic and diverse traffic of user equipment (UE) by clustering the UEs in their respective tracking areas (TAs). Our strategy includes beamforming, which helps reduce energy consumption from the UE side, while SMO minimizes energy use from the BS perspective. We extended our investigation to include \emph{Random}, \emph{Epsilon Greedy}, \emph{Upper Confidence Bound (UCB)}, and \emph{Load Based} sleep mode (SM) strategies. We compared the performance of our proposed C-MAB based SM algorithm with those of \emph{All On} and other alternative approaches. Simulation results show that our proposed method outperforms all other SM strategies in terms of the $10^{th}$ percentile of user rate and average throughput while demonstrating comparable average throughput to the \emph{All On} approach. Importantly, it outperforms all approaches in terms of energy efficiency (EE).
Md Halim Mondol
mmondol@ncsu.edu
Faculty Sponsor: Edgar J. Lobaton
Project Title: AI-Driven Innovations in Chemoinformatics: Predicting VOC Sensing and Molecular Interactions with VAEs and LLMs
Project Abstract
AI-Driven Innovations in Chemoinformatics: Predicting VOC Sensing and Molecular Interactions with VAEs and LLMs
Artificial Intelligence (AI) is transforming chemoinformatics by providing new ways to predict chemical reactions and molecular interactions. This poster explores how AI, especially Variational Autoencoders (VAEs) and Large Language Models (LLMs), can predict volatile organic compounds (VOCs) that dyes can detect. These predictions are based on SMILES, a system for representing molecular structures.
VAEs are a type of deep learning model that helps us understand how molecules interact. By training these models on large datasets like the ZINC database, which contains millions of SMILES strings, we can find complex patterns in chemical data. This ability is crucial for predicting chemical reactions and interactions accurately and quickly, speeding up the discovery process in chemistry.
In addition to VAEs, we use LLMs, which are usually applied in language processing, to interpret and generate SMILES strings. These models help us understand potential reactions and interactions, making them a valuable tool in chemoinformatics. By combining VAEs and LLMs, we can predict molecular behaviors that are hard to understand using traditional methods. One exciting application of this AI approach is creating dye-based sensors that detect VOCs. These sensors are important for industries concerned with air quality and detecting hazardous chemicals. Our research uses AI to predict which VOCs specific dyes can sense, considering factors like the dye matrix, which can affect detection. These predictions help us design sensors with better sensitivity and selectivity for specific uses.
Using AI, especially VAEs and LLMs, in chemoinformatics not only improves our understanding of molecular interactions but also speeds up the development of practical applications. This research shows how AI can offer new solutions to complex problems in chemistry and materials science. Accurately predicting chemical interactions opens the door to innovations like more efficient catalysts and targeted drug delivery systems.
Enrique Pena Martinez
eepena@ncsu.edu
Faculty Sponsor: Michael Kudenov
Project Title: Enhancing Peanut Pod Sizing and Tracking Using Instance Segmentation Techniques
Project Abstract
Enhancing Peanut Pod Sizing and Tracking Using Instance Segmentation Techniques
Peanut (Arachis hypogaea) pod sizing is a critical post-harvest activity within grading stations to determine the grade and value of the harvest. However, the inherent sizing inaccuracies of conventional peanut pod pre-sizer machines have not been thoroughly evaluated. Novel computer vision and low-cost high throughput scanning systems present an opportunity to evaluate pod sizing error, enable pod tracking, and improve pod phenotype data acquisition. In this study, we employed Mask R-CNN and developed a custom tracking algorithm to assess the sizing accuracy of conventional methods. Our model achieved average precision (AP) scores between 0.87 and 0.90 across all pod sizes, providing more consistent sizing predictions across different cultivars compared to the pre-sizer. Additionally, our tracking algorithm successfully maintained track ID association across frames. These results indicate that further integration and development of these technologies could modernize pod sizing processes and increase grading efficiency.
Mushfiqur Rahman
mrahman7@ncsu.edu
Faculty Sponsor: Ismail Guvenc
Project Title: Reinforcement Learning for UAV-Assisted Coverage Hole Detection in Wireless Networks
Project Abstract
Reinforcement Learning for UAV-Assisted Coverage Hole Detection in Wireless Networks
Deployment of the wireless network in a city area is challenging. The complex building structures and their positions create many coverage hole areas with insufficient signal reception from base stations. In this work, we propose a system of coverage hole detection in an outdoor environment setting using a UAV that flies at a fixed altitude and attempts to reach nearby coverage holes. We employ Reinforcement Learning, which takes input from the local building map, the current location of the UAV, and the signal strengths measured by the UAV while searching for coverage holes. The algorithm outputs a 2D array, where the highest value of the array indicates the next optimal location for the UAV. The goal is achieved when a coverage hole is reached. Our experimental results show that proposed method outperforms compared baselines and advances toward theoretical upper bound.
Ishita Pai Raikar
ipairai@ncsu.edu
Faculty Sponsor: George List
Project Title: Media representation of ethical and social issues inherent in Autonomous Vehicle technology
Project Abstract
Media representation of ethical and social issues inherent in Autonomous Vehicle technology
Successful implementation of Autonomous Vehicle (AV) technology is not only an engineering challenge but also a social, political, and ethical one. As AVs become commonplace and begin affecting people’s daily lives in a more profound way, media coverage of the social and ethical considerations of these technologies will follow suit. We seek to analyze and categorize the media’s portrayal of the social and ethical issues surrounding AVs to better understand how these issues are shaping public debate. Through our efforts, an understanding of these impacts will help inform AV system decisions, not only for vehicle manufacturers, but transport system planners and operators as well. Issues that are most salient— defined by frequency, location, and/or length of coverage in the media—become part of the public discourse and influence public policy. Headline-grabbing events in the development of AVs (e.g., an Uber AV fatality or a Tesla auto-pilot accident) increase public discussion and concern about AV technology risk and safety. Moreover, the rise in “fake news” is exacerbating these tendencies and complicating the public dialogue. Our study is a contribution to study public visibility to enhance product development and implementation of AVs which will be steered by public discourse.
Nirjhor Rouf
nrouf2@ncsu.edu
Faculty Sponsor: Paul Franzon
Project Title: Can Low-Rank Knowledge Distillation in LLMs be Useful for Microelectronic Reasoning?
Project Abstract
Can Low-Rank Knowledge Distillation in LLMs be Useful for Microelectronic Reasoning?
In this work, we present empirical results regarding the feasibility of using offline large language models (LLMs) in the context of electronic design automation (EDA). The goal is to investigate and evaluate a contemporary language model’s (Llama-2-7B) ability to function as a microelectronic Q\&A expert as well as its reasoning, and generation capabilities in solving microelectronic-related problems. Llama-2-7B was tested across a variety of adaptation methods, including introducing a novel low-rank knowledge distillation (LoRA-KD) scheme. Our experiments produce both qualitative and quantitative results. Furthermore, we release our evaluation benchmark along with the code necessary to replicate our experiments at https://github.com/FinAminToastCrunch.
Chinmay Savadikar
csavadi@ncsu.edu
Faculty Sponsor: Tianfu Wu
Project Title: GIFT: Generative Interpretable Fine-Tuning
Project Abstract
GIFT: Generative Interpretable Fine-Tuning
We present Generative Interpretable Fine-Tuning (GIFT) for parameter-efficient fine-tuning (PEFT) of pretrained Transformer backbones, which we show can be formulated as a simple factorized matrix multiplication in the parameter space or equivalently in the activation/representation space, and thus embraces built-in interpretability. For a pretrained layer with weights, our proposed GIFT learns the fine-tuned weights directly from the pretrained weights. The parameters of GIFT can be shared by all layers of the pretrained backbone selected for fine-tuning (e.g., all the Query and Value layers), or can be layer-type specific (e.g., different parameters used for Query and Value), resulting in significantly fewer trainable parameters compared to the layer-specific Low-Rank Adaptation (LoRA) method. We perform comprehensive evaluations on natural language tasks (commonsense and arithmetic reasoning, instruction tuning, and sequence classification) and computer vision tasks (fine-grained classification). We obtain the best performance and parameter efficiency among baselines on commonsense reasoning and instruction tuning using the Llama family of models and on visual recognition benchmarks using Vision Transformers. We achieve comparable performance on other benchmarks. Notably, compared to LoRA, we obtain 5.9% absolute increase in average accuracy with 53.8 times reduction of parameters on Commonsense170k using Llama-3 (8B), and 5.4% absolute increase in the win rate with 4 times reduction of parameters using Llama-2 (7B) during instruction tuning. Our GIFT also obtains a slightly higher win rate on instruction tuning than GPT 3.5 (Turbo 1106). We show the output of the first linear layer is surprisingly interpretable, which can play the role of a token-clustering head as a by-product to localize meaningful objects/parts in images for computer vision tasks. Through ablation studies, we verify the effectiveness of the two linear layer formulation by comparing with more sophisticated parameterization schemes. Our results and code is available at https://savadikarc.github.io/gift.
Abolfazl Shahrooei
ashahro@ncsu.edu
Faculty Sponsor: Derek Kamper
Project Title: Data-driven Prediction of Li-ion Battery Cycle Life
Project Abstract
Data-driven Prediction of Li-ion Battery Cycle Life
Lithium-ion (Li-ion) batteries have become indispensable across various sectors, including consumer electronics, renewable energy storage, and electrified transportation, due to their high energy density and reliability. Ensuring their optimal performance necessitates robust Battery Management Systems (BMS) capable of accurate State of Health (SoH) estimation and Remaining Useful Life (RUL) prediction. SoH is defined as the measure of a battery’s current capacity relative to its original capacity, serving as a key indicator of battery health. This work focuses on the application of machine learning techniques to predict cycle life using the data of a small number of initial cycles. The largest publicly available dataset on Li-ion battery charge and discharge cycling is used and comprehensive pre-processing and feature extraction are conducted on the dataset to enhance data quality and extract meaningful parameters for analysis. Specifically, a combination of Fully Connected Perceptron and Convolutional Neural Networks (CNNs) is employed to predict cycle life based on early cycle data, demonstrating the efficacy of deep learning models in this domain.
Suresh Venkatesh
suresh.venkatesh@ncsu.edu
Faculty Sponsor: Suresh Venkatesh
Project Title: Millimeter-Wave Computational Imaging aided by Physics-Informed Neural Nets
Project Abstract
Millimeter-Wave Computational Imaging aided by Physics-Informed Neural Nets
Millimeter-wave (mm-wave) imaging and sensing systems, operating within the frequency range of 30 GHz to 300 GHz, play a pivotal role in a wide array of applications. These include security screening, remote sensing, electronic warfare, and biomedical screening. By harnessing computational imaging techniques, these systems facilitate precise sensing and automatic object detection through the analysis of received or scattered RF signals from the target objects. Nevertheless, despite their remarkable capabilities, these systems encounter significant challenges stemming from noise, dynamic physical environments, and interference from clutter. Traditional methods attempt to address these challenges by increasing the number of measurements and relying on hardware calibrations. However, this approach imposes limitations on overall throughput and escalates system costs. Our approach involves the development of a machine learning (ML)-enabled approach to mm-wave computational imaging. With this approach, we aim to learn and predict these dynamics, enabling the imaging system to adapt robustly to image, visualize, and detect objects in intricate scenes. Such an intelligent imaging system should be proficient at sensing complex environments, adaptively programming the forward channel to illuminate non-line-of-sight (NLOS) targets, estimating propagation effects, and leveraging a priori information from other modalities to reconstruct the region of interest. Using AI/ML based methods, we propose to answer the following questions. 1) Can physics-informed neural nets (PINNs) systematically learn an RF channel on-the-fly? 2) Can PINNs based methods allow for robust and efficient inversion of such RF channels and allow for RF image reconstructions? 3) Can these methods influence the systematic design of sparse RF imaging systems?
Ethan Wilson
eowilson@ncsu.edu
Faculty Sponsor: Chau-Wai Wong
Project Title: FFederated Learning Nodes Can Reconstruct Peers’ Image Data
Project Abstract
Federated Learning Nodes Can Reconstruct Peers’ Image Data
Federated learning is a privacy-preserving machine learning framework that enables multiple clients to train a shared model without sharing raw data. For example, a collection of hospitals could train a model to identify the presence of disease from CT scans without disclosing sensitive medical data. However, this framework does not guarantee client privacy protection. Prior work has shown that the gradient-sharing strategies in federated learning can be vulnerable to data reconstruction attacks. These attacks assume that client gradients are inverted from the system’s central server. In this work, we show that client data is also vulnerable to attacks from other clients, which presents a severe security risk to these systems. We demonstrate that clients can use updates to the shared model weights during federated training to meaningfully reconstruct images from other clients. Additionally, we leverage state-of-the-art diffusion models to improve these reconstructions to a high level of accuracy both at the pixel and semantic level. To the best of our knowledge, we are the first to show that gradient inversion attacks can be carried out by clients in a federated learning framework. By demonstrating the feasibility of this type of attack, we aim to facilitate the development of more robust defenses for federated learning systems.
Jongha Woo
jwoo6@ncsu.edu
Faculty Sponsor: Ning Lu
Project Title: AI-Based Grid Impedance Prediction and Automated PI Gain Tuning for Solar Power Controllers Using the Feed-Forward Neural Network
Project Abstract
AI-Based Grid Impedance Prediction and Automated PI Gain Tuning for Solar Power Controllers Using the Feed-Forward Neural Network
The integration of renewable energy sources, particularly photovoltaic (PV) systems, into electrical grids presents unique challenges, particularly due to fluctuations in grid impedance. These fluctuations are more frequent in distribution networks or micro-grids, where lower voltage levels make the system more susceptible to changes caused by line faults, the inrush of induction motors, or rerouting of power lines. Precise prediction of these impedance changes is crucial for the optimal performance of solar power controllers, which depend on accurate tuning of their proportional-integral (PI) gains.
This research proposes an AI-driven approach utilizing the Levenberg-Marquardt algorithm to predict grid impedance and automatically tune the PI gains of solar power controllers. The core methodology involves measuring the d-axis and q-axis currents and voltages at both the input and output of the PV inverter. These measurements are used to estimate the grid impedance dynamically. The Levenberg-Marquardt algorithm, known for its effectiveness in handling nonlinear optimization problems, is applied to this data to model grid impedance variations accurately.
The AI system then adjusts the PI controller parameters in real-time, ensuring that the PV system operates efficiently across a range of grid conditions. This automation reduces the need for manual tuning, enhancing system stability and minimizing power losses. Simulation results indicate that this AI-based approach significantly improves system performance compared to traditional fixed-gain controllers. The method not only achieves precise impedance predictions but also adapts to varying grid conditions, ensuring optimal controller settings at all times.
This study contributes to the advancement of smart grid technologies by offering a novel solution for the automated tuning of PV system controllers. The use of the Levenberg-Marquardt algorithm provides faster convergence and effectively manages complex, nonlinear relationships within the control system. By integrating advanced algorithms within the control loop, this research demonstrates the potential of AI to optimize the performance of solar power systems, contributing to the stability and sustainability of the electrical grid.
Yifan Wu
ywu66@ncsu.edu
Faculty Sponsor: David Roberts, Alper Bozkurt
Project Title: AI to Predict Aptitude of Candidate Guide Dog Puppies
Project Abstracts
AI to Predict Aptitude of Candidate Guide Dog Puppies
Guide dogs provide tremendously valuable support for people with visual disabilities in every aspect of their lives. Training a successful guide dog can be extremely challenging and costly due to limiting factors, such as the experience level of staff, lack of objective dog evaluation measures, and unpredictability in breeding outcomes. In collaboration with Guiding Eyes for the Blind (GEB), one of the most established non-profit guide dog schools in the US, we are developing and deploying AI to address these challenges. Our approach leverages objective data collected from wearable sensors to predict the aptitude of candidate Guide Dog puppies to enable quantitative metrics for guide dog evaluation. This approach could save donation-funded non-profit guide dog schools like GEB millions of dollars annually.
Guide dog puppies undergo a temperament test at approximately 7.5 weeks of age before entering the socialization and training programs at GEB. Using a sensor-enabled “”smart harness”” system comprising an inertial measurement unit (IMU) and electrodes for electrocardiogram (ECG) measurements, and a custom data aggregation and cataloging software package, GEB staff have recorded and annotated data with more than 500 candidate puppies. Our efforts have included evaluating the accuracy of scoring puppy temperament using the IMU and ECG data that achieves 94% accuracy across 29 dimensions when compared to human expert scoring [3].
To further enhance the capabilities to study candidate guide dogs, we have developed machine learning approaches to reconstruct ECG signals using commodity IMU sensors [1,4].The ML methods are able to accurately separate the ballistic movement of cardiac contraction from body movement. By leveraging IMU sensors to additionally characterize cardiac activity, we can apply these powerful prediction methods on less expensive hardware systems that don’t require specialized staff trained to collect data. This in vivo capability can dramatically broaden these capabilities.
From the age of two months to 14-18 months, each guide dog puppy is placed with a volunteer raiser away from the guide dog organizations to start basic socialization and training. As the raisers are not generally training experts, school professional staff have traditionally relied on their own periodic assessment and qualitative behavior questionnaires completed by volunteer raisers to evaluate the puppies. We have developed a sensor-enabled smart collar device to collect both behavioral and environmental data to further enable in vivo objective remote guide dog assessment. This is achieved through unsupervised learning and location-based statistical analysis to provide detailed behavioral insights to guide dog school professionals [5].
Using this smart collar sensor system, we have also analyzed dog gait by collecting data in various environments to train deep learning models for objective gait analysis [6], which is an important factor during both the training and handler matching processes. After the puppies return to the guide dog organizations, they enter the In For Training phase, a less controlled environment where actual guide dog training begins. The collar sensor collects multimodal data including ambient light, audio, humidity, barometric pressure, temperature, and acceleration. Using this, we have explored using long short-term memory networks (LSTMs), autoencoders (AEs), and kernel principal component analysis (KPCA) to identify behavior patterns and determine the optimal subset of sensor data for predicting temperament [2].
Collectively, these projects combining smart harnesses and smart collars with novel AI capabilities and applications have helped with canine behavior analysis and provided guide dog organizations with an objective, quantitative and efficient way of improving their training and selecting successful guide dogs. These efforts have recently expanded to other schools in Canada and Australia.
Kaixiong Zhou
kzhou22@ncsu.edu
Faculty Sponsor: Kaixiong Zhou
Project Title: TAI-powered IRES Engineering for Compact Size and High Translation in Human Cells
Project Abstract
AI-powered IRES Engineering for Compact Size and High Translation in Human Cells
mRNA therapeutics hold promise in a broad range of medical appli- cations due to the easiness to rapidly and cost-effectively alter RNA sequences for specific treatments. However, standard linear mRNAs are quickly degraded in cells and therefore difficult to achieve a clinically meaningful dosage. Recently, circular RNA (cir- cRNA) has emerged as an intriguing solution due to its prolonged lifespan. circRNA can produce therapeutic proteins from an RNA el- ement called internal ribosome entry sites (IRESs); however, they suffer from low translational efficiency, particularly when consider- ing the size limitations of circRNA. In this work, we leverage syn- thetic biology, high-throughput big data generation, and deep learning to develop novel synthetic IRESs that are compact in size and achieve high translation efficiency in human cells.
Feng Zhu
fzhu5@ncsu.edu
Faculty Sponsor: Aritra Mitra
Project Title: Towards Fast Rates for Federated and Multi-Task Reinforcement Learning
Project Abstract
Towards Fast Rates for Federated and Multi-Task Reinforcement Learning
We consider a setting involving N agents, where each agent interacts with an environment modeled as a Markov Decision Process (MDP). The agents’ MDPs differ in their reward functions, capturing heterogeneous objectives/tasks. The collective goal of the agents is to communicate intermittently via a central server to find a policy that maximizes the average of long-term cumulative rewards across environments. The limited existing work on this topic either only provide asymptotic rates, or generate biased policies, or fail to establish any benefits of collaboration. In response, we propose Fast-FedPG – a novel federated policy gradient algorithm with a carefully designed bias-correction mechanism. Under a gradient-domination condition, we prove that our algorithm guarantees (i) fast linear convergence with exact gradients, and (ii) sub-linear rates that enjoy a linear speedup w.r.t. the number of agents with noisy, truncated policy gradients. Notably, in each case, the convergence is to a globally optimal policy with no heterogeneity-induced bias. In the absence of gradient-domination, we establish convergence to a first-order stationary point at a rate that continues to benefit from collaboration.
Industrial and Systems Engineering
Nafi Ahmed
nahmed3@ncsu.edu
Faculty Sponsor: Rohan Shirwaiker
Project Title: AI-Driven Digital Manufacturing Framework for Biofabrication
Project Abstract
AI-Driven Digital Manufacturing Framework for Biofabrication
Biofabrication, which integrates biological sciences with advanced manufacturing, is vital for innovations in regenerative medicine and cellular agriculture applications. A significant challenge in this field is ensuring that processes are consistent, scalable, and adaptable for rapid translation. This research introduces a new framework for integrating digital manufacturing into biofabrication, using the power of AI to address these challenges. Digital manufacturing enables comprehensive monitoring, precise prediction, and effective optimization, driving robust biofabrication processes. The proposed framework emphasizes data acquisition and analysis through sophisticated sensors that capture biological, material, and process data and demonstrates advanced modeling techniques for biofabrication using both traditional methods and AI tools such as Support Vector Machines (SVM), Neural Networks, Regression Trees, and Ensemble methods to refine biofabrication process parameters. These AI tools are particularly powerful in handling the complex, nonlinear relationships inherent in biological systems. Therefore, these tools are employed to predict outcomes based on variable process parameters, allowing for the fine-tuning of conditions to meet specific biological and mechanical specifications. The framework is demonstrated using a case study focused on optimizing the 3D bioplotting of polycaprolactone (PCL) scaffolds. Insights derived from the digital manufacturing framework guide decisions regarding bioplotting parameters and post-processing. The resulting PCL scaffolds exhibit the desired mechanical integrity and biological compatibility, which are critical for promoting cell proliferation and achieving higher printing resolution. This approach optimizes biofabrication outcomes by integrating physical and biological models and promotes scalability and reproducibility, significantly accelerating the research and development phase. This work underscores the potential of AI-driven digital manufacturing in biofabrication, paving the way for accelerated advancements in cellular agriculture and tissue engineering.
Vasuki Garg
vgarg5@ncsu.edu
Faculty Sponsor: Osman Ozaltin, Maria Mayorga
Project Title: Investigating Illicit Massage Businesses using Image Analysis & Interpretable Models
Project Abstract
Investigating Illicit Massage Businesses using Image Analysis & Interpretable Models
Illicit massage businesses (IMBs) present a significant challenge in the United States by operating under the guise of legitimate massage services while engaging in illegal sex work. This project explores an innovative approach to identifying IMBs through image analysis and risk scoring, aiming to enhance detection and regulatory efforts.
Our study involved analyzing images from two sources—StreetView (Google Streetview) and Yelp (crowd-sourced local business review site) — of massage businesses in Colorado. To address variability in image quality, we developed a heuristic to standardize the StreetView images.
We used the Google Cloud Vision model to annotate objects and text within these images. A frequency analysis of the features extracted from these images identified significant disparities between illicit and non-illicit businesses. These differentiating features were then used as inputs for training an interpretable risk score model.
The risk score model was formulated as a Mixed-Integer Nonlinear Programming (MINLP) problem, aiming to quantify the likelihood of a business being illicit based on the identified features. The optimization process utilized the Lattice Cutting Plane Algorithm (LCPA), which efficiently handles the nonlinear and discrete nature of the problem by recursively splitting the search space and approximating the loss function linearly at each node.
The findings demonstrate that image analysis can effectively identify discriminating features, and when combined with a sophisticated risk-scoring approach, can create accurate prediction models to differentiate between illicit and legitimate massage businesses. This methodology provides a robust and interpretable tool for stakeholders involved in regulatory and enforcement actions, as evidenced by comparisons with baseline models. For future work, expanding the dataset will be crucial for refining the model’s accuracy and generalizability, thereby enhancing efforts to combat illicit activities in the massage industry.
Temilola Gbadamosi-Adeniyi
tgbadam@ncsu.edu
Faculty Sponsor: Tim Horn, Scott Ferguson
Project Title: Enhancing Anomaly Detection in Electron Beam Powder Bed Fusion Additive Manufacturing Using Conv1D Autoencoders and OCSVM-Controlled Real-Time Monitoring
Project Abstract
Enhancing Anomaly Detection in Electron Beam Powder Bed Fusion Additive Manufacturing Using Conv1D Autoencoders and OCSVM-Controlled Real-Time Monitoring
This study explores the application of a one-dimensional convolutional neural network (Conv1D) autoencoder for anomaly detection in the additive manufacturing of Tungsten, utilizing Total Electron Emission (TEE) time series data, with a specific focus on Electron Beam Powder Bed Fusion (EB-PBF) processes. By analyzing localized data defects, the Conv1D model effectively detects both severe and non-severe porosity defects within build layers. Our controlled experiments demonstrate the model’s high efficacy, achieving a 92% recall rate—vital for minimizing the incidence of undetected defects—while maintaining 89% precision, thereby managing the critical trade-off between sensitivity and the reduction of false positives in additive manufacturing quality control. Further enhancing its application to real-time monitoring, features extracted from the Conv1D model were integrated with a One-class Support Vector Machine (OCSVM) to construct a control chart, offering a robust framework for ongoing process evaluation and defect management.
Ethan Houser
ephouser@ncsu.edu
Faculty Sponsor: Sara Shashaani
Project Title: Robust Screening and Partitioning for Feature Selection: A Binary Simulation Optimization Problem
Project Abstract
Robust Screening and Partitioning for Feature Selection: A Binary Simulation Optimization Problem
Feature selection is the process of eliminating irrelevant or redundant covariates in a dataset to construct generalizable interpretable predictions. It is an NP-hard combinatorial problem with only heuristic or model-dependent greedy solution methods. To improve robustness, previous work proposed a zero-one simulation optimization by independently replicating model construction and out-of-sample error calculation. Genetic algorithms were used to solve feature selection showing improved robustness compared to existing techniques, albeit being slow, sensitive to hyperparameters, and lacking convergence guarantees. We propose a stochastic binary search method based on nested partitioning informed by an initial rapid screening phase. With new mechanisms for sampling, we propose steps to efficiently reduce the feature space and obtain an intelligent partitioning scheme. Our experiments show that our proposed algorithm finds fewer irrelevant features, is less sensitive to the data size, and is faster than competing methods.
Yongseok Jeon
yjeon@ncsu.edu
Faculty Sponsor: Sara Shashaani
Project Title: Calibrating Digital Twins using Bayesian Optimization: A Root-Finding Strategy
Project Abstract
Calibrating Digital Twins using Bayesian Optimization: A Root-Finding Strategy
With the rapid growth of attention and development in AI, digital twins are also emerging as pivotal technologies for bridging the gap between the real world and virtual reality. Calibrating DT is a critical yet complex task, which is essential for ensuring the fidelity of these virtual models for their real world counterparts. Bayesian optimization (BO), a powerful tool which is generally used to optimize the expensive-to-evaluate function, is commonly employed for DT calibration as well as a wide range of optimization problems. BO is particularly valuable in scenarios where the objective function is black box, complex (e.g., non-convex) or has unknown derivatives (i.e., hard to obtain) such as hyperparameter tuning in machine learning, experimental design, and many operation research problems including DT calibration.
In this research, we discuss a novel root-finding approach within the BO framework, which is specifically designed for DT calibration, with potential applications in scenarios where aligning a low-fidelity model with a high-fidelity one. For example, this approach could be used to calibrate a simplified model (e.g., logistic regression) which would have a better practical benefits like interpretability and affordable computational time, to approximate the performance of the high-fidelity model (e.g. deep neural network) while minimizing the computational effort.
To accelerate the calibration process, we introduce some novel acquisition functions and unique strategies such as bounding the search space. Our numerical results demonstrate this root-finding strategy (in BO) facilitates the optimization process and provides better calibration results over traditional minimization strategies, contributing to broader adoption in AI-driven real-world applications.
Shaozhi Wang
swang226@ncsu.edu
Faculty Sponsor: Renran Tian
Project Title: Applying Behavior Prediction Algorithms to Improve Social Intelligence of Automated Vehicles for Interacting with Vulnerable Road Users
Project Abstract
Applying Behavior Prediction Algorithms to Improve Social Intelligence of Automated Vehicles for Interacting with Vulnerable Road Users
As automated vehicles (AVs) increasingly become a fixture in urban landscapes, they carry the promise of safer and more efficient transportation. Yet, this promise remains only partially fulfilled, particularly concerning the safety of vulnerable road users (VRUs) such as pedestrians, cyclists, and e-scooter riders. These road users are at a significantly higher risk of injury in the event of a collision. Current AVs often struggle to manage the complex and unpredictable nature of human behavior in real-world settings, and they may misinterpret a pedestrian’s intent to cross the street or fail to anticipate the sudden movements of a cyclist avoiding an obstacle. This gap between the current capabilities of AVs and the requirements for enhancing road safety underscores a critical need: AVs must evolve to develop a form of social intelligence to recognize and predict the behaviors and intentions of those around them, responding safely and intuitively. Without this, the full potential of AV technology will remain out of reach, and the goal of safeguarding all road users—especially vulnerable road users—will continue to be an unavoidable challenge.
To bridge this gap, our research team has developed key tools. One such tool is the Pedestrian Situated Intent (PSI) benchmark dataset, which introduces dynamic intent labels and driver reasoning explanations to improve the understanding of pedestrian interactions. Alongside this, we developed pedestrian intention and behavior prediction algorithms with state-of-the-art accuracies. These innovations mark crucial steps toward making AVs more socially intelligent and better equipped to navigate urban environments safely.
My work continues the efforts in the team. Recognizing the limitations of relying solely on benchmark datasets, my research starts at developing more resilient approaches to pedestrian trajectory prediction. I introduced a training strategy that incorporates perception noise to simulate real-world uncertainties. This led to the creation of the Detecting-Tracking-Matching architecture, designed to assess discrepancies between idealized benchmark annotations and real-world detections. Additionally, the Self-Denoising Att-LSTM (SeDAL) model integrates LSTM networks with Attention layers and a denoising component, significantly enhancing prediction accuracy under noisy conditions.
However, focusing solely on pedestrians is not enough. Urban environments are also shared by a wide range of VRUs, including e-scooter riders and cyclists, who present unique challenges due to their varied behaviors. To capture this broader spectrum of VRU interactions, I join the efforts to collect and process over 200 hours of driving data from various U.S. metro areas recording high-fidelity behavior data of all types of VRUs. This dataset, meticulously calibrated and annotated, allows us to reconstruct and predict behaviors of diverse VRUs. Looking ahead, I plan to enhance these models further with BEVFormer structure, integrating spatial-temporal fusion to improve predictive capabilities. I am also planning to add multi-model inputs such as radar coordinates to improve the model’s reliability. By refining these algorithms, we expect to achieve more accurate trajectory predictions, reducing collision risks and enhancing the model’s ability to handle diverse and noisy real-world data.
Materials Science and Engineering
Sina Dehghan
sdehgha2@ncsu.edu
Faculty Sponsor: Martin Seifird
Project Title: Assessing machine learning methods for predicting conformational structure and aggregation of conjugated polymers
Project Abstract
Assessing machine learning methods for predicting conformational structure and aggregation of conjugated polymers
Preparing conjugated polymer (CP) solutions is one of the key steps in organic semiconductor (OSC) device fabrication. Better understanding of CP conformation is important for controlling thin-film structure and device properties. However, we lack a cohesive, quantitative description of these process-structure-property relationships. Machine learning (ML) algorithms can be used to identify the complex, non-linear interactions between molecular structure, solvent, processing conditions and conformation. Multi-task learning can leverage the complementary nature of multiple target variables (e.g. hydrodynamic radius, Rh, and radius of gyration, Rg) with little overlap in shared training data. We have curated a dataset (ca. 800 data points) of molecular structures, processing parameters, and experimental CP conformation measurements from static and dynamic light scattering (SLS, DLS), and small angle neutron and X-ray scattering (SANS, SAXS). We are investigating the effect of substructure- and property-based representations for encoding CP molecular structure, and ways to encode the repeating, stochastic nature of polymeric systems using oligomeric or periodic representations that can capture monomer-monomer interactions. Ultimately, these data and models can serve as a ”warm start” for ML-guided automated experiments in a self-driving laboratory.
Marium Mostafiz Mou
mmou@ncsu.edu
Faculty Sponsor: Donald Brenner
Project Title: Machine Learning Interatomic Potential for Shock-Induced Phase Transitions in BiFeO3-BaTiO3
Project Abstract
Machine Learning Interatomic Potential for Shock-Induced Phase Transitions in BiFeO3-BaTiO3
Ferroelectric materials are essential for advanced energy storage and power generation technologies due to their superior performance over traditional batteries. They offer longer storage durations and can deliver high voltage, current, and power in short bursts. Among these materials, BiFeO 3 -BaTiO 3 presents a promising alternative to lead-based ferroelectrics. The potential for shock-induced phase transitions in this ferroelectric is promising, as these transitions enable rapid energy discharge, making them ideal for such applications. Density Functional Theory (DFT) has been a crucial tool in examining magnetic and ferroelectric properties, but it faces limitations such as high computational demands and difficulty in accounting for temperature and external field effects. To address the limitations of DFT, we are developing a machine learning-based interatomic potential using Equivariant Neural Networks through the NequIP framework. NequIP leverages the E(3)-equivariant convolution architecture to achieve high data efficiency and accuracy with minimal reference data. This approach respects physical symmetries like rotation and translation, allowing for accurate predictions of interatomic forces and energies. Our goal is to achieve DFT-level accuracy while overcoming its limitations, especially for large-scale simulations. To train this machine learning interatomic potential, we are constructing our data from a comprehensive database of first-principles energies, which includes unit cells subjected to various types of stress and defects. For investigating shock-induced phase transitions, we conducted extensive DFT calculations to identify potential phases as a function of strain and used the generated equations of state to determine transition pressures through the common tangent method. Our initial machine learning potential for BaO has yielded promising results, paving the way for future research that will extend to BaTiO 3 -BiFeO 3 .
Abishec Sundar Senthilvel
asenthi5@ncsu.edu
Faculty Sponsor: Prof. Yaroslava Yingling
Project Title: Data Science methods for Materials Discovery: Yingling Research Group
Project Abstract
Data Science methods for Materials Discovery: Yingling Research Group
The Yingling Research Group is at the forefront of leveraging advanced multiscale molecular modeling, materials-by-design approaches, and data science to explore and innovate in the fields of soft, colloidal, biomimetic, and biological materials. Our research spans a broad range of topics, including in silico characterization techniques for predicting material structure, mechanical and optical properties, chemical stability, and the effects of environmental factors, additives, and impurities. We apply data science techniques to analyze and extract insights from complex, heterogeneous materials characterization data, develop methods for materials data fusion and integration, and advance convergence informatics for phosphorus sustainability. A key focus of our work is enhancing data reliability and minimizing errors, particularly in the handling of small datasets through innovative data imputation and augmentation approaches.
Through interdisciplinary collaboration and cutting-edge data science methodologies, the Yingling Research Group is advancing the understanding and application of materials science to address critical global challenges.
Quanpeng (Sam) Yang
qyang27@ncsu.edu
Faculty Sponsor: Yaroslava Yingling
Project Title: Using Data Science Approaches toward Science and Technology Convergence Research
Project Abstract
Using Data Science Approaches toward Science and Technology Convergence Research
The STEPS (Science and Technologies for Phosphorus Sustainability) Knowledge Hub for Phosphorus Sustainability is a cutting-edge example of using data science to advance convergence research in science and technology. This platform integrates and manages diverse datasets from the STEPS Center, one of the NSF (National Science Foundation) STCs (Science and Technology Centers), providing a comprehensive solution for data collection, storage, analysis, and application. We aggregate phosphorus-related data from STEPS and public sources using advanced tools like a customized web scraper. This data is then sorted and enhanced with natural language processing (NLP) and large language models (LLMs), powering AI-driven tools for materials design, phosphorus flow optimization, and data retrieval. The user-friendly interface allows researchers to efficiently interact with the Knowledge Hub, with AI-driven tools like chatbot-assisted queries and paper recommendations further enhancing its utility. Beyond phosphorus research, the Hub exemplifies how data science can drive convergence research across multiple domains, with its methodologies applicable to other scientific fields. In summary, the STEPS Knowledge Hub demonstrates the power of interdisciplinary collaboration and advanced data science techniques in addressing critical global challenges. Through AI integration, semantic data management, and user-centric design, the Hub offers a robust platform for advancing phosphorus sustainability research and serves as a model for other scientific inquiries.
Mechanical and Aerospace Engineering
Alex Boyer
amboyer2@ncsu.edu
Faculty Sponsor: Joseph Manning, Ashok Gopalarathnam, Roland Kays
Project Title: Machine Learning for Wildlife Monitoring and Conservation in Southern Africa using Unmanned Aerial Vehicles
Project Abstract
Machine Learning for Wildlife Monitoring and Conservation in Southern Africa using Unmanned Aerial Vehicles
We are actively developing deep-learning computer vision models for use in conjunction with unmanned aerial vehicles (UAVs) for the purpose of wildlife monitoring and conservation in Southern Africa.
Regular surveys of wild animal populations are critical for ecological research and wildlife conservation efforts. Regular and repeatable surveys can provide an indication of how a population is doing over time, facilitate habitat and migration studies, and locate important high-value species. One of the most common and accurate survey methods used in Southern Africa for decades is the aerial survey, typically using small, manned aircraft. Using UAVs for these surveys offers the same aerial survey capability, but without the expense and danger of manned aircraft. Aerial surveys with UAVs provides images which are later manually reviewed for wildlife. These surveys generate enough images that manually reviewing and counting animals can easily take 20 times longer than the aerial flights themselves.
NCSU’s Namibia Wildlife Aerial Observatory (WAO) program has been operating in Namibia since 2017 and performing aerial surveys over the Naankuse Wildlife Sanctuary since 2019. Since its inception the program has focused on productive collaboration between NCSU students from engineering and animal science and local wildlife and ecology experts. A major aim of this program is to build local communities’ capacity to use UAVs in wildlife conservation.
Over the past year we have begun incorporating ML/AI in the WAO project. Currently we are training an open-source object detector neural network, YOLOv8, to detect and identify animals in aerial images, using a training dataset of about 22,000 aerial images collected by the Namibia WAO program. The training data contains 17 different mammal species, ranging in size from warthogs to giraffes. The images are of sufficient resolution, and the animals are potentially small enough, that tiled training and inferencing is used to improve detection accuracy. The model is comparably accurate to human counting and several orders of magnitude faster. It is not yet precise enough for unmonitored inferencing, as it has a high false positive rate, but performs well with human assistance to eliminate false predictions. Inferencing time plus a manual review of the model’s inferences to ensure accurate data is still significantly faster than a fully manual count of every image. Attempts to train the model with hard negative mining to increase the precision are currently underway.
Autonomous detection of animals introduces the possibility of detecting animals in real time as the aircraft is flying. The presence or absence of certain species could be used to alter its flight path to further investigate those animals. For example, a survey aircraft may be programmed to detect and loiter around high-value species (like elephants, rhinos, and lions) to observe their behavior in more detail, and to ignore species of lesser concern. Real time detection also enables the use of UAVs in detecting poachers and assisting anti-poaching teams in their interdiction. Alongside aerial abundance surveys, the potential uses of UAVs to assist with anti-poaching efforts is one of the central research focuses of the WAO program.
Anubhav Halder
ahalder@ncsu.edu
Faculty Sponsor: Farhan S. Gandhi
Project Title: Gross Weight, CG Position, and Rotor Flapping Prediction for a Compound Helicopter using Machine Learning
Project Abstract
Gross Weight, CG Position, and Rotor Flapping Prediction for a Compound Helicopter using Machine Learning
The gross weight (GW), center of gravity (CG), and main rotor loads of a rotorcraft can vary during a mission due to factors like fuel consumption and payload changes in multi-hop operations. Monitoring these variations is critical as they impact flight control and operational safety. Previous research has shown that machine learning (ML) models can accurately predict GW and CG for conventional helicopters where the trim solution (main rotor controls, pedal controls, along with aircraft pitch and roll attitude) is unique at a given airspeed. However, compound helicopters, with control redundancy (more variables to control like thrust from propellers, variable main rotor speed, etc.) allow different combinations of controls and aircraft attitudes to correspond to the same GW, CG, and airspeed. This study investigates the use of ML models to estimate the response variables, which are GW, the longitudinal position of the center of gravity (CG_x), and main rotor cyclic flapping angles (β_1c and β_1s) of a compound helicopter with three redundant controls – main rotor RPM, collective propeller thrust, and stabilator (a movable horizontal tail) angle. Neural Network (NN), Gaussian Process for Regression (GPR), and Support Vector Machine (SVM) algorithms are employed to develop estimation models using supervised learning. Inputs (predictors) include airspeed, redundant controls, main rotor controls, aircraft attitudes, and main rotor torque, selected for their availability through the aircraft Health and Usage Monitoring System (HUMS). The dataset is split into low-speed and high-speed regimes to compare the prediction accuracy and training cost of separate regime models against a combined full-regime model. Model accuracy is evaluated using root-mean-squared-error (RMSE) normalized as a percentage of the average step-size of the response variables. GPR generally provided the highest accuracy, followed by NN and SVM, whereas SVM had the lowest computational training cost, followed by NN and GPR. Separate airspeed regime GPR models showed superior performance in GW estimation (RMSE: 0.7%), with higher accuracy and cost-effectiveness compared to a single full-regime model (RMSE: 1.3%). For CG estimation, GPR showed acceptable performance (separate regime RMSE: 22.5%, single full-regime RMSE: 25%), although the maximum outlier errors increase significantly if a 95% confidence interval is considered. Finally, for 1/rev cyclic flapping angle predictions, SVM estimations, though not superior to GPR or NN, were acceptable and had a significantly lower computational cost. Analysis of predictors involved in high inaccuracy outliers revealed that larger outliers often stemmed from observations with multiple boundary variables. A feature exclusion study further examined predictor importance, showing that, on average, variables like rotor RPM and rotor torque were less influential, though their removal led to performance degradation without cost benefits. These findings underscore the importance of selecting ML models tailored to specific operational requirements, such as GPR for accurate CG and GW predictions and SVM for time-efficient flapping angle predictions. The study also demonstrates the feasibility of using these models with only HUMS data, enhancing the practical application of AI in advanced rotorcraft systems without the need for additional sensors.
Anuj Kumar
akumar35@ncsu.edu
Faculty Sponsor: Tarek Echekki
Project Title: Combustion Chemistry with DeepONets
Project Abstract
Combustion Chemistry with DeepONets
A combustion chemistry acceleration scheme for implementation in reacting flow simulations is developed based on deep operator nets (DeepONets). The scheme is based on a mapping of a subset of the thermochemical scalars’ vector between incremental and adaptive time steps. The subset corresponds to variables that are adequate to represent the evolution of the chemical system. The DeepONet-based scheme also relies on a mapping of the solution of this subset onto a corresponding solution at an adaptive time increment to overcome the restrictive requirements of integrating such solutions with stiff chemistry. Training for the DeepONet has also been implemented differently from previous approaches to the solution of PDEs with DeepONets. Instead of constructing solutions from their initial to their final states using DeepONets, the training relies on prescribed short-horizon time windows for training where intermediate solutions also serve as initial states for training. An additional framework of latent-space kinetics identification with modified DeepONet is proposed, which enhances the computational efficiency and widens the applicability of the proposed scheme. The scheme is demonstrated on the “simple” chemical kinetics of hydrogen oxidation and the more complex chemical kinetics of n-dodecane high- and low- temperature. The proposed framework accurately learns the chemical kinetics and efficiently reproduces species and temperature temporal profiles. Moreover, a very large speed-up with a good extrapolation capability is also observed with the proposed scheme.
Arsalan Taassob
techekk@ncsu.edu
Faculty Sponsor: Tarek Echekki
Project Title: A PINN-DeepONet framework for extracting turbulent combustion closure from multiscalar measurements
Project Abstract
A PINN-DeepONet framework for extracting turbulent combustion closure from multiscalar measurements
In this study, we develop a novel framework to extract turbulent combustion closure, including closure for species chemical source terms, from multiscalar and velocity measurements in turbulent flames. The technique is based on a physics-informed neural network (PINN) that combines models for velocity and scalar measurements and a deep operator network (DeepONet) to accommodate spatial measurements and experimental parameters as separate input streams. An additional key innovation is the estimate of the unconditional means of the species’ chemical source terms as additional “observations” to constrain the prediction of these rates. This estimate is based on a convolution of the means of species reaction rates conditioned on principal components of the multiscalar data and the joint probability density functions of these principal components. The PINN-DeepONet method is implemented on the so-called Sydney flames, where training is carried out on 3 and validated on 4 flames. The results show that, despite the limited samples of experimental parameters, including the inlet flow and the fuel jet recess length within the air flow, the PINN-DeepONet approach can construct velocity and scalar fields along with important closure terms for turbulent transport and reaction rates.
Pouria Zaghari
pzaghar@ncsu.edu
Faculty Sponsor: Jong Eun Ryu
Project Title: Application of ML-based Self-directed Online ANN Optimization in Electronic Packaging Through-via Co-design
Project Abstract
Application of ML-based Self-directed Online ANN Optimization in Electronic Packaging Through-via Co-design
Copper-filled via is a critical component of advanced electronic packaging technologies. Embedded in interposer substrate, vias provide enhanced electrical performance in 2.5D and 3D electronic packaging by allowing smaller form factor. In addition to electrical characteristics of an electronic package, its thermal and mechanical performance also depends on via geometry and the interposer material. This necessitates a co-design approach integrating thermal, mechanical, and electrical multiphysics considerations. This research focuses on a parametric study and multi-objective machine learning-based optimization of through-silicon via (TSV) and through-glass via (TGV). This study investigates the multiphysics effects of aspect ratio (AR) and pitch in the square and hexagonal array vias. Copper protrusion, thermal resistance, and electrical parasitics were used as the optimization performance indicators. An online artificial neural network (ANN) algorithm, as well as the conventional genetic algorithm (GA), were adopted to optimize the through-via designs. The parametric study demonstrated that glass substrates are more effective in reducing copper protrusion and mutual capacitance up to 47.5% and 67.6% compared to silicon. However, TSVs showed superior thermal performance. A higher aspect ratio (AR) helps minimize the copper protrusion for mechanical performance. Moreover, the thermal performance was enhanced by reducing the pitch and using hexagonal array vias. Regarding electrical performance, a high pitch and low AR are preferable to minimize electrical parasitics. Finally, a 61.3% decrease in the computation time was achieved by using an online NN-based optimization scheme compared to GA, highlighting its potential in the optimization of high-fidelity complex electronic designs.
Nuclear Engineering
Jonathan Crozier
jpcrozie@ncsu.edu
Faculty Sponsor: Ayman Hawari
Project Title: Deep Learning Methods for Nuclear Reactor Multi-Physics Analysis
Project Abstract
Deep Learning Methods for Nuclear Reactor Multi-Physics Analysis
When fast (i.e., ~2 MeV) neutrons born from fission equilibrate (i.e., thermalize) to the order of kT (where k is the Boltzmann constant and T is temperature), their de Broglie wavelengths and energies approach the order of inter-atomic spacing and elementary lattice oscillations (phonons), respectively. Bragg and phonon scattering alter the trajectory of a thermal neutron in a bound system. Temperature feedback during nuclear transients further impact reaction rates (or cross sections) and the space-time-energy particle spectra near low-lying actinide fuel fission resonances.
The Thermal Scattering Law (TSL), S(a,b,T), where a and b represent energy and momentum exchange between an incoming neutron and the atomic lattice, captures crystalline binding contributions to reaction rates and is proportional to the double differential thermal scattering cross section, with respect to changes in energy (dE–>E’) and scattering angle cosine (mu), as given by the expression below, where (sigma) is the characteristic bound cross section.
(d2sigma / dmu dE–>E’) = (sigma_bound / 2 kT) * sqrt(E’/E) * S(a,b,T)
Typically, evaluated TSL data is discretized and sampled for Monte Carlo (MC) transport. However, a more predictive approach is to store TSL as trained weights and biases for a deep neural network, giving access to the full range of the TSL on-the-fly (OTF) in a MC simulation. AI thus mitigates the need to re-run an expensive phonon expansion calculation for on-the-fly data, and systematically reduces the memory required for high fidelity nuclear data in quickly evolving nuclear scenarios.
With training/validation/test datasets generated from the TSL evaluation code FLASSH (linearized with Pandas and SciKitLearn), deep artificial neural networks (ANNs) for S(a,b,T) data are trained using PyTorch2.0 and 3 NVIDIA V100 GPUs (CUDA11.7) sharing 96 GB of RAM. The ANN backpropagation algorithm uses stochastic gradient descent with the AdamW optimizer. Final average/max prediction accuracy for scattering law data is on the order of .1% / 2% for all a,b,T with good out of sample performance.
Finally, ANNs are serialized as torchscript dictionaries and embedded within Serpent (MC code) using the Python C API to facilitate neural inference OTF. Comparatively, NeTS are more accurate than fitted polynomials, inherently predictive, and more memory efficient by 3 orders of magnitude compared with pseudo-continuous look-up-tables. These methods have been demonstrated for steady-state reactor analysis, the framework is undergoing optimization for implementation in transient multiphysics full-core calculations, which capture the neutronics and thermal-hydraulics of a nuclear reactor. This method outlines a novel approach to coupling modern machine learning with state-of-the-art nuclear reactor analysis.
Junhyoung Gil
jgil@ncsu.edu
Faculty Sponsor: Ayman Hawari
Project Title: AI-Enhanced Nuclear Data Evaluation for Neutron Moderator Analysis in Nuclear Reactors
Project Abstract
AI-Enhanced Nuclear Data Evaluation for Neutron Moderator Analysis in Nuclear Reactors
Advanced Nuclear Reactors are primarily based on concepts that utilize neutron thermalization, within a moderator, to drive the fission reaction in the reactor core. Neutron thermalization is quantified using the Thermal scattering law (TSL), which is essential for predicting the reactor’s operational parameters and criticality. The TSL can be derived from the phonon density of states (DOS) of the moderator material (e.g., light water, graphite, beryllium), which can be calculated through ab initio lattice dynamics (AILD) simulations based on density functional theory (DFT). While AILD offers quantum mechanical accuracy, it is limited to 0 K static environments. Molecular dynamics simulations allow for modelling systems at finite temperatures; however, ab initio molecular dynamics based on DFT is computationally expensive, limiting system size and simulation length, which may introduce accuracy challenges. An alternative approach that combines molecular dynamics with machine learning potentials (MLPs) may provide a viable solution.
This work investigates the application of MLPs to perform machine-learning molecular dynamics (MLMD) simulations, aiming to calculate temperature-dependent phonon DOS of neutron moderator materials with near quantum mechanical accuracy. The Spectral Neighbor Analysis Potential (SNAP) is utilized as the test MLP, and beryllium metal, commonly used as a neutron moderator/reflector, is selected as the test material. SNAP uses data-driven techniques to approximate the potential energy surface of atomic systems by learning directly from quantum mechanical data, and optimizing its hyperparameters through AI-driven methods. The methodology was validated by training the MLP on AILD data for atomic configurations at low temperatures, calculating phonon DOS via MLMD, and benchmarking the results against those from AILD. The study is then extended to higher temperatures, where the trained MLP is used to obtain temperature-dependent phonon DOS.
To date, analysis shows deviations of a few percent between AILD-based DOS and MLMD-based DOS at a low temperature. Because these deviations propagate to the TSL level, current efforts focus on identifying the causes of these deviations to assure the production of accurate nuclear data for advanced reactor analysis and to prevent biases in reactor criticality predictions.”\
Yuqing Huang
hyuqing@ncsu.edu
Faculty Sponsor: Jacob Eapen
Project Title: Superionic-like Diffusion in Yttrium Hydride using Machine Learning Molecular Dynamics
Project Abstract
Superionic-like Diffusion in Yttrium Hydride using Machine Learning Molecular Dynamics
Yttrium hydride (YH2) has emerged as a promising material for the next-generation microreactors and nuclear fission batteries. The mechanism of hydrogen transport, however, remains poorly understood, especially at high temperatures. Our previous study using ab initio molecular dynamics (AIMD) simulations has revelated a correlated diffusive motion of hydrogen atoms in YH2. Due to the high computational cost of AIMD simulations, investigations have been limited to small systems. Recently, machine learning techniques have revolutionized atomistic simulations through the development of rapid and accurate descriptions of potential energy surfaces for diverse materials. In this study, we employ the DeePMD toolkit to develop a deep learning potential for YH2 that is trained using reference data obtained from AIMD calculations on small systems. Classical molecular dynamics simulations are then conducted using this deep learning potential on larger systems with several thousands of atoms for sub and super stoichiometric systems. Our simulations capture and conform the quasi-one-dimensional string-like displacements above 750K with enhanced statistical robustness for YH2. Interestingly, for sub-stoichiometric systems, string-like formation diminishes although with a small activation energy. We rationalize our results from a thermal jamming perspective in close-packed structures that allow superionic-like diffusion in materials.
Operations Research
Yen-Hsi Chou
ychou4@ncsu.edu
Faculty Sponsor: Anderson Rodrigo de Queiroz
Project Title: Convolutional Neural Networks and Transfer Learning Approaches for Enhanced Detection of Rooftop Solar PV Systems in Distributed Power Grids
Project Abstract
Convolutional Neural Networks and Transfer Learning Approaches for Enhanced Detection of Rooftop Solar PV Systems in Distributed Power Grids
Accurate mapping and characterization of distributed rooftop solar photovoltaic (PV) systems are essential for effective energy planning, techno-economics assessment, net demand forecasting, and policy-making. However, traditional methods of gathering data on distributed electricity generation infrastructure are often laborious, costly, or impractical
This study leverages advancements in machine learning from recent years and introduces state-of-the-art image segmentation architectures for automated PV panel detection from remotely sensed data. To enhance performance constrained by training time and dataset size, transfer learning is employed by utilizing pre-trained large-scale deep neural networks as feature extractors. Additionally, a novel hard example mining scheme is proposed as an effective learning framework to further improve model performance.
Employing a comprehensive comparative framework, this study examines a range of factors that influence prediction accuracy, including establishing PV array recognition thresholds, architectural characteristics of image segmentation models, sampling strategies for hard example mining, and strategies for parameter freezing of encoders. The final experimental results demonstrate that the proposed approach achieves superior performance compared to other models in terms of IoU scores, with an F1 score exceeding 0.91 and an IoU exceeding 0.83 in PV panel extraction.
This research contributes to the advancement of automated methods for infrastructure mapping, offering a scalable and efficient solution for monitoring and managing distributed solar PV systems. Additionally, the ideas developed for PV systems detection can be further explored in other areas.
Kuangying Li
kli32@ncsu.edu
Faculty Sponsor: Leila Hajibabai, Ali Hajbabaie
Project Title: Machine Learning-Enhanced Column Generation for a Maximal Covering Location-Allocation Problem
Project Abstract
Machine Learning-Enhanced Column Generation for a Maximal Covering Location-Allocation Problem
This study presents a maximal covering location-allocation problem, formulated as a mixed-integer linear model, aiming to minimize the total cost of vaccine distribution and maximize vaccine allocation to population blocks, while including equity constraints for age, race, and gender groups. A modified Voronoi diagram technique embedded in a Lagrangian relaxation framework is employed to solve the problem. Empirical case studies in Pennsylvania, based on real-world data from the Centers for Disease Control and Prevention (CDC) and health department websites, demonstrate the model’s effectiveness. Numerical experiments indicate that the proposed model outperforms a benchmark column generation technique. However, both methods experience significant CPU times due to extensive data and complex decision variables. To address the computational issue, this study proposes a machine learning-enhanced column generation method that comprises an offline training and an online prediction. The proposed strategy improves computational efficiency while preserving solution quality, effectively reducing runtime, and maintaining an acceptable optimality gap in the location-allocation problem. Preliminary results indicate that the proposed method solves the problem effectively and outperforms solutions obtained from previous work. The enhanced performance results from incorporating predictive analytics within the machine learning process, significantly improving the model’s capacity to manage the complexities associated with integer decision variables.
Jiaxing Wang
jwang97@ncsu.edu
Faculty Sponsor: Dr. Hong Wan
Project Title: GANCQR: Estimating Prediction Intervals for Individual Treatment Effects with GANs
Project Abstract
GANCQR: Estimating Prediction Intervals for Individual Treatment Effects with GANs
Evaluating individual treatment effects (ITE) poses challenges due to the lack of access to counterfactuals, particularly when dealing with biased data. There is a growing interest in harnessing the generative capabilities of Generative Adversarial Networks (GANs) and Variational Autoencoders (VAEs) for ITE estimation. However, few methods effectively address uncertainty quantification of the estimated ITE. In this work, we introduce GANCQR, a GAN-based conformal prediction approach designed to provide prediction intervals for ITE with acceptable coverage. Numerical evaluations on synthetic and semi-synthetic datasets highlight GANCQR’s superiority in handling datasets with selection bias compared to state of-the-art methods.
Teacher Education and Learning Sciences
Shiyan Jiang
sjiang24@ncsu.edu
Faculty Sponsor: Shiyan Jiang
Project Title: Investigation of students’ learning, interest, and career aspirations in an integrated Science and Artificial Intelligence learning environment (i-SAIL)
Project Abstract
Investigation of students’ learning, interest, and career aspirations in an integrated Science and Artificial Intelligence learning environment (i-SAIL)
We are developing and testing an integrated science and AI learning environment in which middle school students will be empowered to create AI solutions to solve science-focused, real-world problems through block-based programming. We investigate the impact of creating such AI solutions in improving students’ learning and cultivating interest and career aspirations. We hypothesize that engaging students in solving science-focused real-world problems that are relevant to them will afford effective learning of science and AI, as well as supporting interest and career aspirations in those fields.